Recently there have been difficulties. I mentioned here back in September a problem that was affecting me at all Wordpress blogs. That was basically due to Akismet and has now gone away. This one is new.
At some stage during this thread, all my comments started going into moderation. At CA that is a semi-ban; moderation can take a day, and it is impossible to engage in any sort of dialogue. But then they started not to emerge at all.
Steve edits firmly at times, in ways that I don't object to. But it's usually transparent. What I do find objectionable is that recent threads have often been quite erroneous, and correction strongly resisted, and now suppressed.
A recent example of error was this, accusing Sven Teske of being a leader of the Nazca vandalism, based on a post of Shub Niggurath. In fact Shub hadn't said that, and clarified his comment. There was no other evidence, but although this was pointed out early (not first by me) there was no response or correction.
I'm writing now about his latest post. It is headed "Important New North American East Coast Proxy Data", and introduces the results of Sicre et al on alkenone analyses off Newfoundland. It suggests that they undermine the results of Marcott et al: "Obviously the Sicre 2014 results provide further evidence against Marcott’s supposed early-20th century blade. At the time, I pointed out that the Marcott blade does not exist in the data and is entirely an artifact of incorrect data handling. To borrow a term from Mark Steyn, the Marcott blade was f……..flawed. It is reprehensible that Marcott and coauthors have failed to issue a corrigendum."
And darkly suggests that they are quietened by the consensus: " Unsurprisingly, the new data was not press released and has thus far attracted no attention."
Well, I read all this and noticed that there were no quotes or references to what the paper actually said. It was based on the archived data and the notes with it. I wondered whether SM had read the paper, which was paywalled. None of the comments seemed to refer to it either.
So I read it, and it seemed to tell a quite different story. The focus of the authors is on the movement of the Labrador Current, which is very cold, and here not so far from the North Atlantic current (Gulf Stream, warm). They spend a lot of time talking about how the LC depends on strength of the NW winds, and may go quite differently to NH SST. The abstract says:
The ice-loaded Labrador Current (LC) is an important component of the western North Atlantic circulation that influences the position and strength of the northern limb of the North Atlantic Current (NAC). This flow of cold and fresh Polar Waters originating from the Arctic has a marked impact on the North Atlantic climate, yet little is known about its variability beyond the instrumental period. In this study, we present the first sub-decadal alkenone-based 2000-year long sea-surface temperature (SST) records from the western Labrador Sea, a climatically crucial region at the boundary between the LC and the NAC. Our results show a clear link between the LC strength and the Northern Annular Mode (NAM), with a stronger NAM and a more vigorous LC during the Medieval Climate Anomaly (MCA). This suggests enhanced LC activity upon future global warming with implications for the Atlantic meridional overturning circulation (AMOC).
So I commented. That comment, "Posted Jan 22, 2015 at 8:51 PM", stayed in moderation for a while, so I thought I would try something that seemed to work earlier, and submitted another version ("Posted Jan 23, 2015 at 1:26 AM") with my name slightly varied, and with a figure. Some time later those both appeared, with a response to the first.
It said, inter alia,
"I presume that you agree that the alkenone SST data indicates substantially warmer mid-Holocene East Coast temperatures than 20th century temperatures. Again, if you wish to argue that this is an expected theoretical outcome and provide references to authors who previously advocated this position"
Odd. I wasn't referring to that at all. Neither were Sicre et al; their results cover just 2000 years. It's in their title. And I was just quoting what they say. So I responded here. This went into moderation, but appeared quite soon. The response:
"Nor is Stokes’ theory of a cold MWP in Labrador consistent with other information discussed here"
Well, it's not my theory. It's Sicre et al, and I had showed their Fig 6. But by now, I was fairly convinced that Steve hadn't read the paper, so I asked:
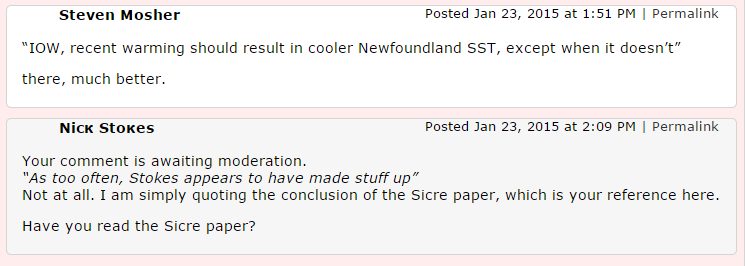
That is still in moderation, three days later. Meanwhile, there was a little further commentary, based on a claim that SST in Placentia bay had not gone down. I had pretty much given up at that stage, but one query by R Graf (above the one shown) seemed addressed to me so I sought to respond:
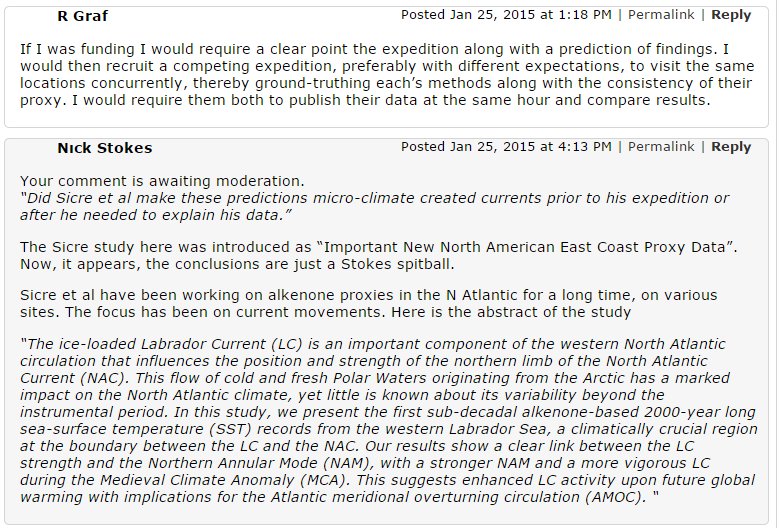
Still in moderation. So I had pretty much lost interest, when I saw that Steve McIntyre had, four days after my first comment, come up with a substantial response. It seems he has finally read the paper. But not allowed my earlier comments.
I'll respond a little here. He says
"Stokes says that Sicre et al “chose sites that are very sensitive to movements in the Labrador Current”. This is either an error or a fabrication in respect to the Placentia Bay site, used in the main comparison with Sachs et al 2007 Laurentian Fan site.
...
Sicre et al explicitly stated that the “SE site” (Placentia Bay) was in the “boundary zone between the Labrador Current and the Gulf Stream”. "
Exactly. The boundary zone is very sensitive to movements.
"Stokes asserts that Sicre et al 2014 postulated an “antiphase” relationship between the NE Bonavista Bay site (in the Labrador Current) and offshore Iceland.
...
There is no observable “antiphase” relationship between the Placentia Bay site and MD99-2275."
Yes. But this data is presented as a contradiction the the Marcott claim that temperatures rose in modern times. Whether antiphase or neutral, it does not provide that contradiction.
Update 11.22AM 27/01 Well, well. I see that my second comment there has now appeared, with a response. The screenshot I showed was timestamped 9.52 AM 27/01, both Melbourne time. The response basically argues that you can like the data without liking what the authors say about it.