The spherical harmonics map is here:
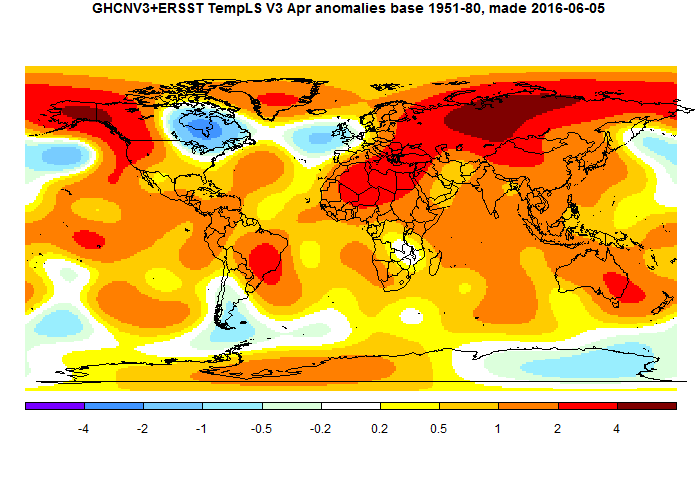
Again unusually warm in Russia and W North America (except Labrador strait region). Not so warm in Arctic, cold over Hudson's Bay.
In the attributions plot, the main point of interest is that SST has risen again. The land pattern is similar to recent, but more subdued.
In other news, JAXA Arctic Sea Ice shows continued melting and record low levels; since mid April the margin between it and previous years has become quite marked.
The PDO continues to be interesting, now taking on its more classic, warm-phase look. The NOAA PDO number ticked up again slightly in April... 6th uptick in a row.
ReplyDeleteJCH, the PDO is too sluggish to make any snap judgements on.
DeleteWhere its at is to make sense of ENSO and maybe this will lead to insight on PDO.
The deal with ENSO is that it's all geophysics and hydrodynamics. Taking the latest interpretation of what is going on, we can look at the differential equation decomposition of the ENSO time series and fit a forcing model to the oscillations.
http://imageshack.com/a/img921/4664/mJFuv4.png
That's essentially four well-known parameters.
http://contextearth.com/2016/05/07/crucial-recent-citations-for-enso/
The arctic melting season is off to a very interesting start. Sea-ice extent and area down, NH snow cover down, temps up, volume near all-time lows for this point in the season, and weather forecasts for more 'big blue' - with insolation growing each day.
ReplyDeleteIf you're not already reading Neven's Arctic Sea Ice Forum: The 2016 Melting Season topic it's the best place to keep abreast of what's happening on a daily basis.
SST has risen again.
ReplyDeleteThere's an interesting annual cycle which has developed over the past decade in global average SST anomalies, with trough around February/March and peak around July/August. I haven't seen anyone comment on this before. The timing of its appearance and magnitude are dependent on baseline choice. Using 1951-1980 it starts to become clear around 2005.
The source is the Northern Hemisphere, where cycle amplitude is currently around 0.3K. Seems to be mostly the Extratropics. CMIP5 models produce this pattern as well, though to varying degrees. GCMs also show an opposite but much smaller cycle in Southern Hemisphere SST anomalies.
I would guess this has something to do with sea ice and/or land-sea fluxes?
That's an interesting observation. I would think there should be some annual cycle (for obvious solar reasons), but you are saying that it is strengthening in recent years?
DeleteYes, it could be cyclic. There is quite a large cycle with that phase in land/ocean absolute temperatures. Land has a big effect there, but the cycle may also be present in SST. So a cycle appearing in the anomalies would suggest that the amplitude is growing a little. That's consistent with the anomaly base dependence.
DeleteI think sea ice is a likely cause. It's very hard to assign a monthly anomaly to water that, at that time, was frozen in the base period. The default is to assign a base period value of -1.8°C (freezing point of sea water). Then any measured SST in current times shows up as a positive summer anomaly.
Yes, it has strengthened. Here are global average SST monthly anomalies with February, March, July, August marked.
DeleteFocusing just on Northern Hemisphere anomalies, the pattern is stronger. I've plotted for 0-90N and 0-60N to show that SST measurement oddities in sea ice areas do not appear to be significant.
Looking just at the Extratropics (25-60N) removes much of the ENSO influence to make the pattern clearer.
And here's the view from the HadGEM2-ES model for NH Extratropics.
That is amazing. I had kind of assumed that the seasonal cycle was compensated out of the time series, but if it is increasing in amplitude like that over recent years, compensating the signal risks severely distorting the underlying behavior. So best to leave it like that.
DeleteThe ENSO signal is locked to both an annual cycle and a metastable biennial cycle, and modulated by at least 2 angular momentum wobbles and 2 long-period lunar tidal factors.
The folowing PNG is a wave-equation-transformed ENSO signal aligned with a nominal forcing model.
http://imageshack.com/a/img921/4664/mJFuv4.png
I suggest there is little chaotic or red noise content to these oceanic behaviors, and the oscillatory pattern is strictly forced by known external factors. My gut says this was not identified because phase reversals can play havoc wrt traditional signal processing analysis approaches.
Around blogs there is this notion that OHC always goes down after an El Nino. I don't think that is always true. OHC did take a big dip after 1998, but is OHC going down now?
ReplyDeleteJCH said:
Delete"Around blogs there is this notion that OHC always goes down after an El Nino. I don't think that is always true. OHC did take a big dip after 1998, but is OHC going down now?"
ENSO is predominately a sloshing of the thermocline layer forced by angular momentum changes in the earth's rotation. Changes in heat content likely occur as the different layers are exposed to the surface.
More remarkable than all that is the fact that ENSO is predictable. Much like tidal analysis, if one enters the parameters corresponding to the Chandler wobble, long-term lunar tidal periods, etc., the resultant time-series response will match the observed ENSO behavior with amazing fidelity.
http://imageshack.com/a/img922/3556/QDCA6E.png
This is the realm of geophysics. We really have to try to understand the rich dynamics of what we have historically measured, instead of chasing incremental trends. If we do that, I guarantee all these other questions on heat content changes will start to get sorted out. You really have to learn to crawl before you can walk.
It would be interesting to know the highest probability reached in ENSO prediction that ultimately failed. A La Nina in the fall is currently at 70%. I wonder if Vegas agrees.
DeleteIndeed, all of these ENSO models fail more than a few months in advance because they assume that the behavior is a stochastic process. If I configured SPICE simulations on an electrical circuit like they do on ENSO models, they would call me nuts. About the only extra consideration is if the circuit showed metastable properties.
DeleteAs a oomparuson, why don't they run stochastic models for tidal prediction? For the same reason -- its nuts to configure a stochastic model for a stationary deterministic outcome. Most of the outcomes will be off.
Do it this way:
http://imageshack.com/a/img923/4327/geUPHO.png
JCH,
Delete"Around blogs there is this notion that OHC always goes down after an El Nino. I don't think that is always true. OHC did take a big dip after 1998, but is OHC going down now?"
Well the NOAA/NODC data for first quarter 2016 is out now. OHC and 0-2000 m global average temps are down slightly from first quarter 2015. On the other hand, data from Argo marine Atlas (Argo only) suggest that OHC and temps continue to rise unabatedly.
(I dont trust climatologies and compare only with the same period earlier years, etc)
I have a personal calculation of OHC based on Argo data only. It tracks NOAA/NODC quite well since 2007 when the ARGO array was fully deployed. The comparison also includes modelled OHC estimated from CMIP5 TOA imbalance. The data, 12 month running means, goes through March 2016.
Olof R - thanks. Time will tell, but I don't expect there to be much of a dip in OHC as this El Nino unwinds.
DeleteOlof R,
DeleteYou should not roule out that there can be a small decline in 0-2000m OHC, you have alway to look at the nature of physics on El-Nino-Events, try this way, if its going to an El-Nino, the trade winds weakens, therefore Upwelling weakens and with this, vertical mixing is weaken. So whats then happen? In easy words, the energy by solare incoming and the radiative imblance caused by humans emissions will be trapt in the upper layer or say in the mixed Layer, just look at the 0-100m of the ocean: http://data.nodc.noaa.gov/woa/DATA_ANALYSIS/3M_HEAT_CONTENT/DATA/basin/3month_mt/T-dC-w0-100m1-3.dat
While the upper ocean is warming, the ocean below the "mixed Layer" should not increase or cool down a bit, because its just more then this, you also should note, that on the polar caps, cool water is sink down and transport in direction to the lower latitudes.
On the other Hand, the Temperature on Surface has risen strong, in the middle latitudes we would lost some Energy by OLR
Taken this together, we should not role out the possibility, that OHC can decrease for a short time under this circumstances
After the 1998 El Nino there was a large drop in OHC. Often scientists talk about EL Nino warmth as coming from within the ocean - a disgorging of energy into the atmosphere. I think this has been a very poor description that has hung on since 1998. It appears to me that OHC usually goes up, on net, regardless of what ENSO does, and that the 1998 drop was an unusual event. If the TOA imbalance is positive, more in than out, talking about OHC fueling El Nino warmth has an obvious problem.
DeleteAnon, I expect a slowdown in the rate of OHC increase during an el Nino. There is a lot of heat diverted to warm the troposphere and ground plus melting of ice, and warmer atmosphere leads larger OLR loss to space.
DeleteThe troposphere has been about 0.5 C warmer the recent winter than the winter before, a rate of change that is 20 times larger than the longterm warming trend. If troposphere and continents in the long run represents 4% of Global heat increase, a twenty-fold increase could almost "steal" all of the ocean warming in one year.
Also, the Arctic sea ice volume is 1700 km3 lower now compared to one year ago. That is about 5% of the annual Global long-term heat increase. The Antarctic sea ice volume is also much lower this year, the change may reach similar numbers as the Arctic ice. And then there is the (probably) increased OLR.
Thus, there shouldn't be much heat left to warm the oceans. NOAA/NODC report a slight decrease (-0.002 C )in ocean temperature 0-2000 m between first quarter 2015 and 2016. It is surprising that Argo Marine Atlas show an increase of +0.008 C for the same period, and virtually no sign of slowdown in the recent OHC increase. Doesn't Argo data make up more than 90% of the input for the NODC OHC analysis?
Considering all the fuel needed to for El Nino warmth and energy losses, can there be an extra energy source that keeps the OHC increase unabated as Argo Marine Atlas suggests. For instance water vapour feedback, increased greenhouse effect...?
The boring answer may very well be that the differences between NODC and Argo Atlas is within error bounds.
Finally, I have a chart
showing the difference in Ocean temps between the recent October-March and last years Oct -Mar, with data from Argo Marine Atlas.
There is a large temperature increase in the 0-100 m layer, a substantial decrease between 100 and 400 m, and small increases from 400 down to 2000 m.
I think the amount of sunlight entering the oceans between the two periods needs to be known. I suspect it is roughly equivalent. A roughly equivalent amount has to come back out, or the oceans, on net, will either cool or warm. They're warming, so less is coming back out, which is consistent with the energy imbalance. The ocean loses its heat from the cool skin layer. Additional ACO2 is causing it to be bombarded with long wave radiation, which penetrates just a few microns. With less wind, the surface just sits there and takes it. The top 100 meters fills with warm water that is sloshing back across from the Western Pacific. That does not mean that energy leaves the oceans; in most El Nino events it's just stored in a different place: roughly, the SST measurement area. The cool skin layer is laying on top of a warmer water column. This increases evaporation. I don't see anything in this that is extra.
DeleteJHC at 8:47 AM,
DeleteI would wonder, if warming is coming from the ocean itself, because tropical oceans are also under El-Nino cooler below the mixed layer, that what the surface is warming if more that, upwelling of cool water is less and the upwelled water itself is warmer then usually (because of Downwelling Kelvinwaves)
In climatology, the pacific tropics are a heat sink, if the heat sink is reduced we get El-Nino
And Yes, under the line, El-Nino doesent matter, all whats matter is imbalance, El-Nino-Cycle only influence the noise in the uptakte itself.
Olof R at 8:02 PM,
Delete"Finally, I have a chart..."
Yes, that is what El-Nino is, as said yesterday, below the mixing layer, the heat sink is reduced, so therefore, in climatolgy there is usually heat sink, so if its not like due el-nino, sharp below the mixed layer, the water should be getting cooler, while the mixed layer itsef becomes much warmer.
To the Argo Marine Atlas, have to look for myself, i found Marine Atlas is a little bit tricky, so therefore my answer on this comes later
Olof R,
DeleteSo found +0.0053K for Ja-Ma in compare to Ja-Ma 2015, but i think it really because of that marine Atlas has not the equal area to NODC-Data.
Just watch out the difference between 70N-70S and 90N-90S based on NODC:
https://climexp.knmi.nl/data/inodc_heat2000_0.50-359.50E_-70-70N_n_mean1_anom_30.dat
https://climexp.knmi.nl/data/inodc_heat2000_0.50-359.50E_-90-90N_n_mean1_anom_30.dat
You see, the last few years, in 70N-70S was mostly arround 0.01K warmer then 90N-90S
In my opinion, this should explain the difference
Anon,
DeleteI have downloaded the NOAA/NODC OHC anomaly netcdf and done som checking now.
I believe that Argo Marine atlas only covers 65N to 65S but there are only a few percent of the total ocean volume outside these bounds.
Differences in the polar seas does not explain the cooling in NODC OHC, on the contrary both polar seas have been warming.
The change in OHC between first quarter of 2016 and 2015 is: (unit 10^22J)
65N-90N +0.07, 65N-65S -0.65, 65S-90S +0.09, Globally -0,49
(My integration of OHC differs slightly from the official numbers, that have a Global -0.54)
This makes the difference even more mysterious. Can a small share of extra measurements with other methods than Argo really change the outcome?
I also wonder, How common and reliable are temperature measurements in the ice covered polar seas. Arctic buoys can read temps down to 100 m, but deeper profiles must be sparse. Is OHC in polar seas mostly based on extraploation from profiles near the ice edges?
BTW, you are right about +0.0053 C for Argo Marine Atlas. The 0.008 that I hastily picked wrong was something else, ie the recent one year change in 12m-running means.
PS, NOAA/NODC data for OHC and ocean temperatures was updated through March today at Knmi climate explorer.
DeleteGlobal Ocean Temperatures 0-2000m, 12-month running means, show a slowdown in the recent three months, but no dip
http://climexp.knmi.nl/data/inodc_temp2000_0-360E_-90-90N_n_mean12_12v_anom_30.png
Strangely, the OHC calculation from the same provider, show a decrease for the last three months.
http://climexp.knmi.nl/data/inodc_heat2000_0-360E_-90-90N_n_mean12_12v_anom_30.png
Same data, different results. Is there something special with the Levitus method?
Now there are two methods against one suggesting that the OHC hasn't decreased during the recent el Nino..
So can we safely call into doubt ENSO descriptions suggesting that during the hiatus/pause/slowdown energy was sequestered in the oceans and that it has come back out of the oceans during the recent El Nino, which has officially slipped into neutral territory? Energy was sequestered in the oceans during the pause/hiatus/slowdown, and it's still sequestered in the oceans. The energy that has caused all these recent record monthly GMST anomalies was caused by the interactions of 2015 and 2016 sunlight with the earth systems, one of which is its control knob, ACO2 at 400+ppm.
DeleteNo, I have to disagree (with JCH). It is a mistake to compare 1st qtr 16 to 4th qtr 15. Better to compare Y-O-Y changes. OHC was down slightly from 1st qtr 15 to 1st qtr 16, after several years of strong increases (23.417 down to 22.874 * 10^22 J for 0-2000m). It was 11.9 ten years ago, and 5.03 twenty years ago, so the average rate of accumulation is twice as fast in the last ten years vs previous decade.
Delete0-100m ocean temps were way up, due to this El Nino. 100-700m temps were way down. ENSO just exchanges the heat from deep to shallow ocean depths and back again. Higher temps at the surface can then vent some of the heat to the atmosphere (and then to space). But the long term change is more and more heat accumulating, and the rate is accelerating.
The issue with ENSO and El Nino is that the first item of business for geophysicists is to nail the standing wave phenomenon that describes the behavior. Until they can do that it is all empirical hand-waving and heuristics used to disguise the shortcomings in understanding the underlying physics. Worse yet is that you have theorists like Tsonis, who incidentally joined the AGW-denying GPWF as a committee member recently, claiming that ENSO is largely chaotic and so is immune to detailed analysis -- apart of course from what Tsonis himself deems as important.
DeleteBut if you ignore what Tsonis says and just do the physical time-series analysis assuming that known forcing causes the non-autonomous oscillations, then we may in fact be able to get a handle on the dynamics of ocean-heat content transfer. The key is to model the sloshing of the thermocline as it sets up its standing wave pattern. Once you fit to the driving periods, then one can infer the amount of deeper cool water exposed to the surface. I don't think GCMs are required to do this because the effect can ignore all the faster and higher wavenumber details.
Heck if this is not all straightforward geophysics and solving a simple wave equation:
updated PDF Sloshing Model for ENSO
Nick, seeing contour maps always reminds me how I like to see bubble plots of the color-coded values for the individual weather stations superimposed to show the local variability and spatial coverage. I don't recall ever seeing anyone do this for global temperature anomalies, but I think it would be a much better display, ideally mapped onto a zoomable globe for better viewing of station-dense areas. The bubble plus contour approach allows a better assessment of actual data coverage (at least for land stations) and local variability compared to the contour values alone.
ReplyDeleteWhen I look at monthly temperature anomalies for NWS stations in Texas, I typically see a spread of 1C or more just in the tiny geographic area of Texas.
Bryan,
Delete" I don't recall ever seeing anyone do this for global temperature anomalies, but I think it would be a much better display, ideally mapped onto a zoomable globe for better viewing of station-dense areas."
My preferred way of doing that is with a triangular mesh. There is color shading for temperature, organised so that the color is right at each station/node and shaded in between. The plot is on a sphere, with trackball facility for viewing, and it is zoomable, and also clickable for detail. You can ask to see stations and mesh. There is an example here for monthly GHCN/ERSST, which is a by-product of TempLS and is kept up to date. There is another example here for trends, also maintained.
I think it does have the good features you describe - it shows local variability as well as pattern.
Thanks Nick. I like that approach, especially with the option to display the stations and mesh. It's also great to see it on a moveable and zoomable globe. Excellent work. It really shows how sparse the land coverage is in many areas. I noticed for April 2016 you can see a lot of variability in the US where the station density is high. I can imagine most land surfaces would look like that if we had good coverage. I also noticed that Australia has hardly any stations. Why is that?
DeleteThe station density in Canada is appallingly low considering how many surface stations are reporting data in real-time. I also noticed China was totally missing as you reported.
Bryan,
DeleteThanks. Yes, Canada and Australia are rather sparse. GHCN V3 in recent times uses the data that countries submit monthly to the WMO on CLIMAT forms. You can see this data collected here. I suppose countries differ as to how much effort they are prepared to put into this, though I suspect Canada and Australia are limited by the number of good stations in sparsely populated areas.
A few years ago there were interruptions to the Canada info when they were not supplying some of the necessary information (normals etc). One of the problems with recent fussing over data errors etc is that the system has become rather rigid. If data like normals are not supplied, but are otherwise known, GHCN won't take the responsibility of fixing it themselves. Same with obvious errors. They just omit the data until the source country submits an amended report.
Nick writes us here: '... Not so warm in Arctic, ...'.
ReplyDeleteMaybe maybe, but certainly not in what UAH/RSS understand under 'lower troposphere' above the North Pole region.
Below are their NoPol TLT anom's for January-April 2016.
- UAH 5.6: 3.16 2.00 2.22 2.41
- UAH6.0beta5: 2.44 1.51 1.44 1.89
- RSS3.3: 3.30 2.11 1.44 2.23
Wow wow.
Estimating from TempLS GISS April may be 1.13°C. Using this value, I update my prediction for GISS LOTI 2016 to 1.08+-0.08°C.
ReplyDeleteSounds reasonable to me. I might have thought a little higher in April.
DeleteYes, why not 1.20 C? Anyway, I find it very likely that Giss April becomes the third warmest monthly anomaly ever..
DeleteThe 1.13°C was calculated by simple linear regression of the time series (from the Monthly global temperature anomaly data at this site)that have already an April 2016 value.
DeleteI redone the calculation with the more sophisticated method, I use for the prediction of the annual temperature, and including annual sin and cos function.
I get 1.16+-0.07°C for the GISS LOTI April 2016 temperature.
Using this value the prediction for the year 2016 changes to 1.09+-0.08°C.
Uli,
DeleteYours turned out to be accurate. It came in at 1.11 °C.
Yes, but this may be by accident. 4 years of monthly data are very little to do the linear regression from TempLS and satellite data. After the last TempLS update (rise in temp) it changes to 1.17+-0.07°C.
DeleteConservation of energy applied in an emergent structures analysis identifies climate drivers. The match between calculated and measured average global temperatures is 97% since before 1900 even when excluding any influence from CO2. Incorporating the influence of CO2 improves the match by 0.1% http://globalclimatedrivers.blogspot.com
ReplyDeleteNope, that's not a strong correlation. You have to be very careful of applying a correlation coefficient calculation to something that is mainly a gradual trend. It's very easy to get correlation coefficients upwards of 0.99 with very little effort. I am afraid that you have missed out on that part of your statistics education.
DeleteDan,
DeleteI note that "measured" is not a consistent index, but made up of a dubious hybrid of HADCRUT and UAH. And the model makes no sense to me. It starts with a fitted A, said to be ocean cycles, and then adds a radiative flux and a CO2 term. It makes some physical sense that the latter might be explanatory for T, but not for the residual after you have already subtracted a fitted term.
But the main problem for CO2 attribution is that the multivariate uncertainties are not calculated. The model effectively relates, T, A, sum(T) and sum(log(CO2)). All except A have rather similar exponential growth, which means the regression cannot well distinguish the coefficients.
@whut – It is pure fantasy to say “It’s very easy to get correlation coefficients upward of 0.99 with very little effort”. The near-perfect correlation I obtained is especially remarkable when correlating with anything in the natural world where the ‘measured’ trajectory contains multiple uneven up and down trends. Not only that, but the ‘match’ is for R^2 which is the square of the correlation coefficient. An R^2 of 0.97 = a correlation coefficient of 0.9849. No one else has achieved anywhere near this excellent explanation of the average global temperature trajectory since before 1900.
DeleteMany good engineering textbooks explain the first law of thermodynamics. Wikipedia has an article on Coefficient of Determination. Read up and then try it yourself.
Nick – It appears that you have gotten mired in minutia. It is not a regression in the usual sense. It is a stand-alone equation that calculates an average global temperature (AGT) trajectory that is an excellent match to trajectory measurements. The method used to refine the influence coefficients and proxy factor might be new to you. A profound discovery is that CO2 has no significant effect on climate.
A measure of the validity of a predictive equation is its ability to predict. In an earlier paper at http://agwunveiled.blogspot.com I calibrated the equation using data to 1990 and predicted the AGT trajectory from there. That prediction is within 0.03 K of the smoothed measured AGT for 2010.
The agencies reporting surface temperatures have committed science malpractice in changing their measured data in attempt to corroborate a global warming agenda. The distortion caused by the on-going super El Nino which is sure to be followed by an equally dramatic La Nina further complicates things. My best estimates of reality are Figure 12 (no CO2) and Figure 13 (includes effect due to reported CO2).
"The agencies reporting surface temperatures have committed science malpractice in changing their measured data in attempt to corroborate a global warming agenda"
DeleteThis is arrant nonsense, and makes the rest not worth reading. What are you matching if not the reported surface temperatures? It seems just stuff you have made up.
"The agencies reporting surface temperatures have committed science malpractice in changing their measured data in attempt to corroborate a global warming agenda"
DeleteWell, in my world it would be malpractice not to do the ship-buoy SST bias correction, when you know that the bias is there.
GHCN v3 algorithms also regularly cool arctic stations, from Svalbard eastward through Alaska, by 1-2 C. The recent Winter this has not been enough , GHCN has simply removed 5 months of data from two Russian stations with anomalies of more than +10 C, despite the fact that this persistent hotspot is corroborated by reanalyses etc.
"A measure of the validity of a predictive equation is its ability to predict"
There might be oceanic 60-year cycles, but they are easily removed by a 60-year smooth. Here is my instant model for climate prediction
If I still live in 2036, I would be very surprised if the Hadcrut4 60-year average wasn't at least 0,5 C. What is your prediction?
Dan,
DeleteReading off your Figure 6, it looks like you are predicting a temperature for 2020 to be about 0.34, which is about 0.2 lower than the average for 1998 (to make it easier to determine your baseline offset), with a +/- 2.5 sigma range of 0.1 to 0.55 C.
If 2020 temps end up outside your 2.5 sigma range, will you concede your curve fitting exercise has no predictive power?
Are you willing to place a bet with real money?
Using just a regression of ln(CO2), I predict 2020 will be higher than 1998 by 0.23 C +/-0.2, so 0.78 using your baseline, with a range of 0.58 to 0.98 C. Since our ranges do not overlap, it should be clear who is right (unless it falls in between).
All that remains is to determine the temperature index to use. I am willing to use an average of GISS, NOAA, HadCrut, RSS and UAH. Sound good to you?
Dan is likely a frustrated engineer with an agenda.
DeleteLike Nick says, dealing with a relatively secular trend like that, you only have one factor that you can play with, any more and they become undistinguishable. For example, if you try to include two factors, they will work as a difference pair to create nonsense results -- one will likely be large and positive and the other large and negative as it tries to fit the curve. In physics, that's what's called a degenerate solution.
The agenda-revealing aside is that Dan both complains about the quality of the data, and then says he can fit to some incredible correlation. Again Nick was spot on in suggesting that he can't have his cake and eat it too.
So Dan, if you want to try to put on your big-boy engineering pants, why don't you try modeling the oscillations of ENSO over the course of 100 years? See how high a correlation coefficient you can get on that monster.
Nick – So you choose to keep the blinders on and continue to allow yourself to be deceived.
DeleteOut of curiosity, I used the method with the latest HadCRUT4 reported data and several other temperature data sets and even usED Leif Svalgaard’s final version of ‘new’ sunspot count. All (except Figure 10) resulted in R^2 of 0.97 or better.
Olof – I see you have the rationalization for changing the data down pat.
Mother Nature does not take directing so the tinkering with data does not alter the truth. My method is robust. Best assessment of the true AGT results in R^2 of 0.97 or better as demonstrated by latest HadCRUT4 data set.
All measured AGT data sets since before 1900 match a 64 year net of ocean cycle effect, not a 60 year cycle. My predictions for the trend depend on what happens with solar cycle 25. My eyeball estimate of average of Hadcrut4 temperatures 2007-2036 is a bit lower at about 0.3 K. Note that my 1990 prediction, described above, of 2010 trend temperature was within 0.03 K.
Will – Figure 12, which uses smoothed data, is a bit better than Figure 6. I agree with comparing things by magnitude of change rather than the values themselves.
The method has already demonstrated its ability to predict as described above.
As to betting on the future reported temperatures, the only thing meaningful would be the trend because of the apparently random fluctuations in reported data with equivalent s.d. of about 0.09 K. The huge effective thermal capacitance dictates a time constant of about 5 years. On top of that, there is no way to tell how desperate the various agencies will become to continue to corroborate their global warming agenda. The growing separation between surface and satellite-based AGT data sets 2011-2015 is indicative. The exaggerated response of the satellite-based data sets to the on-going super El Nino is typical.
Although I am convinced the AGT trend is down, about the only way to demonstrate it, other than my analysis, is by reduced growing season and crop failure. Because the downtrend is only about 0.18 K per DECADE, I doubt I will be around long enough for that to become widely recognized.
Oh my, I can get correlation coefficients that high easily!
DeleteUsing CSALT model
I use a ln(CO2) factor and a crooked LOD factor for long-periods (the "crookedness" of the LOD component is not degenerate with the monotonic ln(CO2) ). The rest are oscillatory components that match the ENSO cycles and impulse responses that capture volcanic responses.
I am not saying that this model is any great shakes. I will refer to my model of ENSO for that, which is based on first principles physics.
ENSO model
Although the correlation coefficient is much less in the ENSO model than the CSALT model, it is explainable because there is NO secular trend in the ENSO time-series that will "artificially" inflate the correlation. That's what a secular trend does -- it fools you just as Feynman would say :)
Dan, if you don't get this intrinsic property of correlations, then there is no hope for you.
"I used the method with the latest HadCRUT4 reported data and several other temperature data sets and even usED Leif Svalgaard’s final version of ‘new’ sunspot count. All (except Figure 10) resulted in R^2 of 0.97 or better."
DeleteSo HADCRUT 4 must be pretty good then?
Nick, It does not cater to Dan's agenda to fix the obvious flaw in his argument. To his audience, just-so stories trump logical reasoning.
Delete@whut: yes, he's a mechanical engineer (MSME) who works in the automotive sector and has been blogging & commenting about climate for eight or nine years. The usual tedious mixture: CO2 has an insignificant effect on climate, CO2 is plant food, RW gun proponent, socialist conspiracies, blah blah blah. So climate change is due to rascally climate elves or the sunspots or both.
Deleteyup, deniers such as Dan are so predictable. They invariably tend to only look for cycles of 50 to 100 years in period because those are the only scales that could possibly benefit them in trying to debunk AGW. And that's why they like to integrate behaviors such as sunspot counts, as the integration step will act as a low-pass filter and bring out the slower cycles. They do this even though it doesn't make any sense, as they are desperate for explanations, no matter how far-fetched they sound to us.
DeleteOTOH, what is nice about their misguided focus is that the field is wide open to try to understand the faster time-scale variability. Dan could care less about that because it doesn't help build his preposterous arguments.
Nick – All that I have looked at are ‘pretty good’ but they are also all corrupted by the unsmoothed spike of the on-going super El Nino which is sure to be compensated for by a super La Nina which is quite sure to follow.
DeleteWhut – Thanks for the warning that the ENSO model graph is bogus. I had seen it before and expected as much.
Perhaps you have not realized yet that, since my equation stems from the first law of thermodynamics, your comments are not relevant. Also, the huge effective thermal capacitance of the planet, which contributes to a time constant of 5 years, easily demonstrates that the csalt results are nonsense and rules out the significance of “faster time-scale variability”.
Given your demonstrated bias and/or lack of broad engineering science skill this and the detailed explanation in the paper and references might not be grasped. It will be interesting to see just how wide the growing separation between the rising CO2 and not-rising average global temperature will need to get before you realize that perhaps you missed something.
Dan, What exactly are you talking about now?
DeleteIn reality, what we are faced with when it comes to ENSO modeling is dealing with scientists such as AA Tsonis. Tsonis essentially claims that ENSO is chaotic and cannot be predicted, and since he has a paper in the prestigious PRL on the topic, people think he has credibility.
Well, I just found this news about the Tsonis. Within the last few days he joined the anti-climate science Global Warming Policy Foundation as a council member.
This may be the tipping point in terms of Tsonis' scientific credibility. Unless he has been living under a rock, Tsonis had to have known that this organization is purely political and has no interest in advancing science. Like many of these organizations, they decide on a name that only makes it sound like they are interested in the science.
There are many different problems with Dan Pangburn's analysis. Nick mentions some above. I'd like to comment on them in more detail.
DeleteHe's calculating correlation coefficients between pairs of smoothed time series -- the sawtoothed "oceans" term effectively is a massively smoothed version of the PDO, and the temperature data are smoothed over 5 years.
Then there's the business of summing (accumulating) the annual sunspot and CO2 terms. Dan is comparing temperature (on a 5-year average) with infinitely accumulated sunspots and CO2. This implies that the temperature in year Y would be 100X more affected by sunspots in year Y-100 (a century earlier) than in year Y itself. And of course accumulating annual CO2 concentrations is even more bizarre, since the concentrations are themselves the result of accumulated emissions of CO2 over time.
For the sunpsots, this accumulation process "works" for Dan because it turns the otherwise useless sunspot data into a monotonically rising time series that is artificially more correlated with the also-rising temperature data. The r2 value for non-accumulated sunspots vs annual global mean temperature is about 0.015 (from 1895-2015). Accumulating the sunspots, and turning them into a monotonically rising series, balloons that to 0.80, and then using the 5-year smoothed temperatures inflates it further to 0.87.
In other words, just for the sunspots, Dan's methods have effectively inflated the r2 value 50-fold. Impressive!
Yet another problem is that Dan's interpretation of his results is totally wrong. He has a model that uses a hand-picked sawtooth pattern (claimed to represent "ocean cycles") plus his 105-year accumulated sunspots, and the result is claimed to be correlated with temperature at an r2 of 0.97. Dan interprets this as meaning that ocean cycles and sunspots explain 97% of the temperature trend, and that "all other factors" (including CO2) "must find room in that unexplained 3%".
BUT THAT INTERPRETATION IS TOTALLY WRONG. If you use an even simpler model (natural log of CO2 vs 5-year average temperatures) the correlation approximately 0.90 ... which by Dan's own interpretive process would mean that CO2 alone explains 90% of the variation in temperatures, and that "all other factors" including ocean cycles and sunspots and anthropogenic aerosols and other greenhouse gases and volcanoes and everything else "must find room in that remaining" 10%.
So do ocean cycles and sunspots explain 97% of the variation in temperature? Or less than 10%?
Dan's method of interpreting his results -- calculating an r2 and assuming that all terms omitted from the model cannot explain more than (1-r2) of the variation in the dependent variable -- would result in his believing two wildly contradictory things.
Accumulating sunspots ad infinitum turns the sunspot data into a monotonically rising series. CO2 concentrations since the late 1800s are also monotonically rising. You can compare either one to temperatures (which are also mostly rising) and get a very high r2 value. Dan's lack of understanding of statistics leads him to ascribe a meaning to that r2 value that is simply wrong.
See below for minor correction.
DeleteDan has obviously never visited Spurious Correlations
ReplyDeleteDid you know that US spending on Space, Science and Technology from 1999-2009 correlates with Suicides by Hanging, Strangulation, and Suffocation at 99.79%!!!!
Kev – Do you not recognize that your observations have nothing to do with an equation applying the first law of thermodynamics which calculates a 97% match with measurements for at least 120 years?
DeleteDan - The point is that high correlations are dime a dozen contradicting your claim. And you realize that denying CO2's role in warming defies physics, don't you? When you write your Nobel Prize winning paper that overturns a century or more of radiate transfer and fundamental physics I'll be all ears. Until then it's will be one LOL after another. Time has taught me that serious responses to nutters are a waste of pixels and energy.
DeleteAnyone who argues against correcting for known biases should be asked if they've ever had to set a clock.
ReplyDeleteCorrecting known biases improves accuracy. It’s the unrecognized and/or denied biases that lead to mistakes.
DeleteGreat, then people should stop criticizing NOAA, NASA, Hadley/CRU etc. for improving the accuracy of their products
DeleteThere are many different problems with Dan Pangburn's analysis. Nick mentions some above. I'd like to comment on them in more detail.
ReplyDeleteHe's calculating correlation coefficients between pairs of smoothed time series -- the sawtoothed "oceans" term effectively is a massively smoothed version of the PDO, and the temperature data are smoothed over 5 years.
Then there's the business of summing (accumulating) the annual sunspot and CO2 terms. Dan is comparing temperature (on a 5-year average) with infinitely accumulated sunspots and CO2. This implies that the temperature in year Y would be 100X more affected by sunspots in year Y-100 (a century earlier) than in year Y itself. And of course accumulating annual CO2 concentrations is even more bizarre, since the concentrations are themselves the result of accumulated emissions of CO2 over time.
For the sunpsots, this accumulation process "works" for Dan because it turns the otherwise useless sunspot data into a monotonically rising time series that is artificially more correlated with the also-rising temperature data. The r2 value for non-accumulated sunspots vs annual global mean temperature is about 0.015 (from 1895-2015). Accumulating the sunspots, and turning them into a monotonically rising series, balloons that to 0.80, and then using the 5-year smoothed temperatures inflates it further to 0.87.
In other words, just for the sunspots, Dan's methods have effectively inflated the r2 value 50-fold. Impressive!
Yet another problem is that Dan's interpretation of his results is totally wrong. He has a model that uses a hand-picked sawtooth pattern (claimed to represent "ocean cycles") plus his 105-year accumulated sunspots, and the result is claimed to be correlated with temperature at an r2 of 0.97. Dan interprets this as meaning that ocean cycles and sunspots explain 97% of the temperature trend, and that "all other factors" (including CO2) "must find room in that unexplained 3%".
BUT THAT INTERPRETATION IS TOTALLY WRONG. If you use an even simpler model (natural log of CO2 vs 5-year average temperatures) the correlation approximately 0.90 ... which by Dan's own interpretive process would mean that CO2 alone explains 90% of the variation in temperatures, and that "all other factors" including ocean cycles and sunspots and anthropogenic aerosols and other greenhouse gases and volcanoes and everything else "must find room in that remaining" 10%.
So do ocean cycles and sunspots explain 97% of the variation in temperature? Or less than 10%?
Dan's method of interpreting his results -- calculating an r2 and assuming that all terms omitted from the model cannot explain more than (1-r2) of the variation in the dependent variable -- would result in his believing two wildly contradictory things.
Accumulating sunspots ad infinitum turns the sunspot data into a monotonically rising series. CO2 concentrations since the late 1800s are also monotonically rising. You can compare either one to temperatures (which are also mostly rising) and get a very high r2 value. Dan's lack of understanding of statistics leads him to ascribe a meaning to that r2 value that is simply wrong.
Minor correction: What Dan accumulates ad infinitum is the annual difference between Sunspot Number and 34 (i.e., sunspots minus 34), not sunspot number itself.
DeleteThis still turns the sunspot time-series into a nearly-but-not-quite monotonically rising series.
This results in the following changes to the correlations I mentioned in the above comment:
Annual sunspots with annual temperatures: 1.51 [no change]
Accumulated sunspots with annual temperatures: 0.79 [was 0.80]
Accumulated sunspots with 5-year temperatures: 0.86 [was 0.87]
This does not change any of my conclusions. Dan's methods are still non-physical.
Yet another comment (Nick, sorry about spamming your blog here...)
DeleteThe 3rd paragraph of my comment above ("Then there's the business of summing ...") is not entirely correct.
For the versions of Dan's model where F=0 (e.g., Figure 6, the source of his "r2 = 0.97" claim), a sunspot in year Y-100 will have exactly the same effect on temperatures in year Y as would a sunspot in year Y-50 or year y-1. In other words, a transient warming or cooling of the planet will raise or lower its temperature uniformly forever.
The larger issues remain. Dan's interpretation of r2 values is fundamentally wrong. Using Dan's method of interpreting r2 values, sunspots and ocean cycles account for either 97% or less than 10% of the measured temperature change. CO2 accounts for either less than 3% or 90%. It's not meaningful at all.
Ned W – The analysis is correct.
DeleteI assume you looked, although your apparent lack of engineering science skill resulted in failure to grasp it. Your comparison of “non-accumulated sunspots vs. average global mean temperature” is a nonsense comparison of a measure of power with a measure of energy. Your statement “infinitely accumulated” indicates you apparently don’t even understand the math.
Apparently you are unfamiliar with the method. It reveals the comparative influence of the factors considered. CO2 alone makes a good correlation but the time-integral of sunspot number anomalies makes a better one so it prevails; nearly extinguishing any influence of CO2.
CO2 increase from 1800 to 2001 was 89.5 ppmv (parts per million by volume). The atmospheric carbon dioxide level has now (through April, 2016) increased since 2001 by 33.5 ppmv (an amount equal to 37.4% of the increase that took place from 1800 to 2001) (1800, 281.6 ppmv; 2001, 371.13 ppmv; April, 2016, 404.6 ppmv).
The average global temperature trend since 2001 is essentially flat. The near flat-line of the temperature trend with increasing CO2 corroborates that CO2 has no significant effect on climate.
The validity of any model is in its ability to predict. The method/equation allows prediction of temperature trends using data up to any date. The predicted temperature anomaly in 2010 calculated using data to 1990 is within 0.03 K of the smoothed measured trend.
Read up on engineering thermodynamics (especially the first law) and transient heat transfer analysis and you might be able to understand what is going on. Study up on radiation heat transfer, thermalization and the rudiments of quantum mechanics and you might begin to understand why.
"The validity of any model is in its ability to predict."
DeleteSo here is your prediction. Big cooling starting 2005. It isn't looking good.
Big cooling starting 2005??? Well, it looks really bad...
DeleteIt will take a lot of "creative" engineering thermodynamics to hide the bulk of global warming
This chart represent about 80% of the global warming, with an additional 13% going deeper than 2000m, and 7% melting ice, heating the ground and atmosphere(only 1-2%).
Since 2005 the 0-2000 m ocean layer has accumulated 12.2*10^22 J, which with the assumption that this represents 80% suggests a total global energy imbalance of about 0.95 W/m2 during the 2005-2015 period.
BTW, with a simple linear regression I can get an r of 0.997 (r2 = 0.994) between Hadcrut4 60-year running means and historical CO2 levels.
Comments on Dan's latest, part 1:
DeleteDP: "I assume you looked, although your apparent lack of engineering science skill resulted in failure to grasp it."
I did look, first casually skimming it and then going back over it in more detail. To put it kindly, your work is a mess. For example, your model turns out to be extremely sensitive to the value of the term Savg, which you describe as being the average sunspot number but actually functions as a threshold above which the model has earth gaining energy and below which it is losing energy. Different values for Savg will radically change your results. You pick a value of Savg = 34 sunspots. In one place (following eq. 1) you state that this is the approximate average sunspot number 1610-1940, but your source (ref 3) for sunspot numbers only goes back to 1700, and the actual average up to 1940 is 44, not 34. Later (after fig. 5) you provide a different justification for the choice of Savg = 34, stating that it is based on the period 1895-2015, but the actual average value for this period is approx. 59, not 34.
This kind of thing makes it difficult to reconstruct exactly what you're doing and why you're doing it.
DP: "Your comparison of 'non-accumulated sunspots vs. average global mean temperature' is a nonsense comparison of a measure of power with a measure of energy. Your statement 'infinitely accumulated' indicates you apparently don’t even understand the math."
The model used in your figure 6 just assumes that 34 sunspots represents the dividing line between earth gaining and losing energy. An additional sunspot in the year 1900 will raise global temperatures by the same dT value *forever* -- dT will be the same in 1901 as in 2001 or 3001. The temperature in 2016 will be affected equally by an extra sunspot in 1915 or 2015.
In other words, you cite Schwartz for a 5-year time constant, but your model actually has an *infinite* time constant.
DP: "Apparently you are unfamiliar with the method. It reveals the comparative influence of the factors considered. CO2 alone makes a good correlation but the time-integral of sunspot number anomalies makes a better one so it prevails; nearly extinguishing any influence of CO2."
No, this is not how to interpret a correlation coefficient. You are claiming that if two series, X1 and Y, have a correlation coefficient of r, then all other potential factors [X2, X3, ... Xn] combined cannot explain more than 100*(1-r^2) percent of the observed variation in Y. But that claim is trivially easy to disprove!
I computed your version of the global surface temperature series, by aligning and averaging HADCRUT3, HADCRUT4, NOAA, and GISS. I plotted it on your graph to verify that it matched. For your period of 1895-2012, the correlation of this temperature series with the natural log of CO2 has an r2 of 0.85. The correlation with your "sunspots minus 34, times 0.003553, summed, and divided by 17" is 0.78.
Based on the way you interpret r2 values, CO2 explains 85% of the variation in temperatures 1895-2012, meaning that everything else explains only 15%... AND, simultaneously, sunspots explain 78% of the variation in temperatures, meaning that everything else explains only 22%. Those two interpretations, both of which follow from your method, are mutually contradictory. Therefore, your method of interpreting r2 values is wrong.
The problem is obvious and straightforward. Can you figure it out?
and further comments:
DeleteDP: "CO2 increase from 1800 to 2001 was 89.5 ppmv (parts per million by volume). The atmospheric carbon dioxide level has now (through April, 2016) increased since 2001 by 33.5 ppmv (an amount equal to 37.4% of the increase that took place from 1800 to 2001) (1800, 281.6 ppmv; 2001, 371.13 ppmv; April, 2016, 404.6 ppmv). The average global temperature trend since 2001 is essentially flat. The near flat-line of the temperature trend with increasing CO2 corroborates that CO2 has no significant effect on climate."
The radiative forcing from CO2 is proportional to the natural log of concentration; it's not linear. In radiative forcing, the increase from 371.1 to 404.6 ppmv is 31% of the increase from 281.6 to 371.1, not 37%.
And the global temperature trend since 2001 is not flat -- in your own temperature time-series (average of HADCRUT3 + HADCRUT4 + NOAA + GISS) the trend is +0.13 K/decade from 2001-2015. So even your cherry-picking of 2001 in an attempt to minimize the recent warming fails.
DP: "Read up on engineering thermodynamics (especially the first law) and transient heat transfer analysis and you might be able to understand what is going on. Study up on radiation heat transfer, thermalization and the rudiments of quantum mechanics and you might begin to understand why."
It's cute that you can use big words like "thermodynamics" and "quantum mechanics," but you do realize that your model is just a cartoon, right?
Behind all the big words, your model is just taking a hand-picked 64-year sawtooth oscillation and adding to it a weird transformation of numbers of sunspots (i.e., sunspots minus 34, times 0.003553, summed annually, and then divided by 17).
There's no physics; it's just a bunch of manipulations that serve to give the result you want. But the real howler is your claim that an r^2 of 0.97 for a model without CO2 means that CO2 must explain less than 3% of the variation in temperature. The fact that you still keep defending this is hilarious!
Ned (3:02 AM) – You are correct in realizing that Savg is a threshold, but R^2 is not ‘sensitive’ (say ± 25%) to it when looking at R^2 for the period 1895-2012. It might appear sensitive if you do not adjust the coefficients to maximize R^2 for each trial of Savg. See Table 1 in http://agwunveiled.blogspot.com . For SSN 1610-1700 I scaled the NASA graph at http://solarscience.msfc.nasa.gov/images/ssn_yearly.jpg to get the graph I used as shown at Figure 5 in http://globalclimatedrivers.blogspot.com The ‘later’ sentence (after Figure 5) was poorly worded. Replace it with “The highest R^2 over the period 1895-2015 was found with Savg = 34 when coefficients C and F are set to zero.” (Thanks for that, I will fix the blog).
DeleteThe slow change to the temperature trend (0.6 K in 32 years) justifies the much simpler ‘steady-state’ type solution using Equation 1. The 5-year time constant justifies smoothing the measured AGT. Nothing wrong here as far as the overall objective is concerned.
You say “…trivially easy to disprove.” True but not relevant. The objective here is to determine the contribution of CO2 to global warming. The fact that R^2 was not significantly changed whether CO2 was considered or not demonstrates that CO2 has no significant effect on climate.
If you don’t include anything for ocean cycles you get R^2 = 0.78. That is about right (unsmoothed) but why do you ignore ocean cycles? As shown in Table 1, ocean cycles account for about 37% of the temperature change 1909-2015.
The rest of your post indicates that you still do not grasp (or perhaps don’t accept as valid) the method. Looking at each component separately is misleading. Looking at all named factors at once and maximizing R^2 allows determination of influence coefficients (and the combined influence coefficient/proxy factor) of each. As I stated above, this method predicted the smoothed 2010 trend within 0.03 K using data through 1990.
I've already spent more time on this than is really justifiable, so I'll just stick with the most important point here:
DeleteDP: "The objective here is to determine the contribution of CO2 to global warming. The fact that R^2 was not significantly changed whether CO2 was considered or not demonstrates that CO2 has no significant effect on climate."
I'm sorry, Dan, but no. You cannot draw the conclusion you want from this.
Let's say you believe that two factors, X1 and X2, might affect some third variable Y. You build a model to predict Y based on X1 and it has a relatively high r^2 value. Then you add in X2 and it doesn't improve the model fit very much. Your conclusion is that X1 is much more important than X2 as a driver of Y.
But suppose you looked at X2 first, and found it had an equally good (or better) fit when used to predict Y on its own. Then adding X1 into the model didn't do much. You would now conclude exactly the opposite -- X2 is more important than X1.
This is possible because both of the "independent" variables X1 and X2 are actually highly correlated with each other. Either one of them would yield a high correlation with the dependent variable Y. You are picking the one that you like, observing that it's well-correlated with Y, and declaring that the other obviously doesn't matter. Someone else could do the opposite -- point out that CO2 alone has a high correlation with temperature, and conclude that sunspots don't matter.
Let's look at the numbers. I've prepared the following three data sets, based on the material you provided in your work:
T = your global temperature index (average of HADCRUT3, HADCRUT4, GISS, and NOAA)
CO2 = natural logarithm of CO2 in ppmv
SSN = time-integrated scaled sunspot number departure from 34 (SSN minus 34, times 0.003553, summed and then divided by 17)
Here are the correlations:
T and CO2: 0.85
T and SSN: 0.78
CO2 and SSN: 0.92
Basically, what has happened is that your process of manipulating the raw SSNs has turned them into something that looks a lot like the time evolution of CO2 -- rising slowly before 1950, and then faster after. Since CO2 (or LN[CO2]) is highly correlated with temperature, a transformed SSN that looks somewhat like CO2 will also be pretty well correlated with temperature.
That, in a nutshell, is the story of your model.
Ned (5:05 AM) – I am fully aware of the argument that the influence of CO2 declines logarithmically with increasing concentration. What with thermalization, convection and reverse-thermalization, I have doubts as to whether that perception is valid. That is partly why I only talked about concentration. But even 31% is a pretty big number.
DeleteWhere did you get info for HadCRUT3 after 2013?
I see you selected only the agencies that report surface temperatures. The agencies reporting surface temperatures have committed science malpractice in changing their measured data in attempt to corroborate a global warming agenda. The old data thru 2014 is shown at http://endofgw.blogspot.com . There is a growing separation between the surface data and the LT data from satellite sensors starting in about 2011. The separation rate has been fairly steady with the LT trend flat until about Nov, 2015 with the arrival of the on-going super El Nino. I don’t believe that planet energy has been increasing.
Your perception that thermodynamics is a ‘big word’ is revealing. Perhaps that explains some of your antagonism towards Equation 1. It is based on the first law of thermodynamics.
Your lack of understanding of thermodynamics (which is a part of physics you have apparently not been exposed to) helps explain the nonsense in your last two paragraphs.
Continuing from the previous...
DeleteWhat happens if you use multiple regression to estimate T based on both CO2 and accumulated-SSN-anomaly simultaneously? Here are the results:
r^2 = 0.85
t-statistics: CO2 = 7.35; SSN = -0.1
p-values: CO2 = 2.78E-11; SSN = 0.92
coefficients: CO2 = 3.18; SSN = -0.016
In other words, when the two are put together, CO2 is highly significant, while SSN (even in its time-accumulated anomaly form) is utterly insignificant. In fact, the sign of the SSN coefficient is actually slightly negative, meaning that insofar as sunspots have any effect within the model, they are cooling rather than warming ... but basically they have no effect of consequence.
This should not be surprising to anyone. See Grant Petty's textbook on radiation in the atmosphere. Meanwhile, sunspots are only really meaningful as a proxy for solar irradiance, which we can now measure directly, so we know that its decadal variation is small in comparison to the forcing from trace gases.
And that's what's really decisive here -- our knowledge about mechanisms, not the empirical regression models above.
In point of fact, adding in your sawtoothed 64-year oscillation as a third term in the multiple regression model actually changes things enough to make all three terms (CO2, SSN, and oscillation) significant in terms of their p-values (and this time, the signs of the correlations are all going the "right" way).
That's cool! -- but it doesn't actually mean that your manipulations of SSN or your 64-year oscillation are *correct*. Just as with the simple correlations in the first part, you can't draw any conclusions about other non-tested alternatives from this model. Even if X1, X2, and X3 do a great job of predicting Y, it may turn out that X1 and X3 are actually spurious, and only "work" in this context because they superficially resemble the shape of un-tested series X4 and X5, which if we had included them would have led to an even better model.
Here is what I'd conclude with:
* CO2 and other greenhouse gases are an important part of the planetary climate. Trying to prove otherwise is a fool's errand.
* Decadal (and longer) variations in solar radiation are small (in comparison to CO2 etc.) but non-negligible. Rather than using overly complicated manipulations of sunspot numbers, I would recommend using TSI (from direct measurement in recent years, and reconstructed TSI from sunspots for the past). Keep in mind that the TOA fluctuation in TSI needs to be reduced to account for the spherical earth and the earth's albedo.
* Internal variability in the form of ocean-atmosphere cycles does matter at decadal scales. It's not my area of expertise so I won't comment on ways to represent it in these kinds of "toy" models.
Oh, good grief.
DeleteDP: "The agencies reporting surface temperatures have committed science malpractice in changing their measured data in attempt to corroborate a global warming agenda."
Spare us the crap, please. Nobody here is interested in that silly propaganda.
DP: "Your perception that thermodynamics is a ‘big word’ is revealing. Perhaps that explains some of your antagonism towards Equation 1."
No, it was just sarcasm.
"Spare us the crap, please."
DeleteYes, it is crap. I've been chronicling the fumblings of the GWPF panel that was supposed to expose all this, but somehow couldn't find anything to report on.
Land measured data is not changed - you can find it in GHCN unadjusted, essentially unchanged since 1995 (except for new data). It is homogenised for long term averages as it should be, but makes very little difference. And SST adjustment, if anything, has a cooling effect.
And as thermodynamics, your Eq 1 is nonsense. Thermodynamics allows you to equate energy fluxes, as with the radiation and CO2 terms there, and even to a temperature rate, standing in for change of internal energy. But including a purely empirical temperature approx ("ocean cycles") makes nonsense of all that. Just one term destroys any pretence of energy balance.
It's worth noting that insofar as Dan's ostensible goal is to determine the relative importance of CO2 and solar forcings, he's going about it in a very inefficient way. Why mess around with temperature at all? Just calculate the forcings for CO2 and solar and compare them directly, in W m-2. That avoids the need for any kind of debatable empirical conversion from radiant flux to temperature (e.g., ECS or TCS or ESS or Dan's empirically fitted model coefficients).
DeleteCO2 data are available from these sources:
Years 1-2004: https://www.ncdc.noaa.gov/cdo/f?p=519:1:0::::P1_study_id:9959
Years 1959-2015: ftp://aftp.cmdl.noaa.gov/products/trends/co2/co2_annmean_mlo.txt
Reconstructed solar irradiance (TSI) is here:
Years 1610-2014: http://lasp.colorado.edu/lisird/tss/historical_tsi.csv?
Radiative forcing for CO2 is 5.35 * ln(CO2_i/CO2_i0) where ln is natural log, CO2_i is CO2 concentration (ppmv) in year i, and CO2_i0 is CO2 in some reference year.
Radiative forcing for TSI is (1-a)*(TSI_i)/4 - (1-a)*(TSI_i0)/4 where a is the planetary albedo (0.3), TSI_i is top-of-atmosphere total solar irradiance in year i, TSI_i0 is solar for some reference year.
Here is the result, showing forcings relative to 1700.
This is almost a perfect apples-to-apples comparison, in that the source data sets are fairly un-controversial and the conversion to radiative forcing is straightforward. At the margins, it's possible that there would be minor differences in the climate sensitivity to these two different sources of forcing, because their spatial and seasonal distributions are different and thus the relative strengths of various feedbacks would differ. That is a decidedly second-order effect, though.
Ned (1:18 PM) – OK, I figured out what you are talking about regarding the significance of CO2. In my statement “The objective here …”, replace ‘has’ with ‘can have’ and add “Best estimate for the significance of CO2 using available data to date is shown in Table 1 row# M of http://globalclimatedrivers.blogspot.com . Figure 13 is the associated graph showing the anomaly comparisons.
DeleteNed (1:28 PM) – I have only done multiple linear regression analysis (decades ago) so I have no clue what tools are out there. I ran across this as a required condition for doing multiple regression “Assumption #6: Your data must not show multicollinearity, which occurs when you have two or more independent variables that are highly correlated with each other.” at https://statistics.laerd.com/spss-tutorials/multiple-regression-using-spss-statistics.php . It appears that the high correlation between CO2 and SSN would cause a problem.
TSI variation is way too small to directly account for AGT changes so an effect multiplier would need to be present. I don’t know of any for TSI but a possible one has been suggested with SSN as a proxy.
Ned (1:31 PM) & Nick – There is evidence it is not ‘crap’. I have been tracking the data changes. The growing separation between the reported surface data and reported satellite data is corroborative that the surface data has been jiggered.
A lot of paychecks depend on increased CO2 being a problem. It isn’t. For most of earth’s history CO2 level has been several times higher than the present. The planet plunged into the Andean-Saharan ice age when the CO2 level was over ten times higher than now. You can get links to this from my 2008 paper at http://www.middlebury.net/op-ed/pangburn.html
Nick (4:05 PM) –Multiply both sides of the equation by thcap to make it is easier to see that all factors are in terms of energy.
Ned (9:50 PM) – Thanks for the links. I use Law Dome for early, Moana Loa for recent and extrapolate using a 2nd order fit 1980-2012 for CO2 ppmv. I added a graph as Figure 4.5 to show this.
I had not seen the TSI source.
The influence coefficient for CO2 is an output of my analysis, not an input. I got a value of 0.188 compared to your 5.35.
It doesn’t work to do a different analysis, get a significantly different AGT from mine and claim mine is wrong. The only thing that is proven is that one of us is wrong. Right now AGT is corrupted by the on-going super El Nino. It will be revealing to see the AGT when its influence dies out. I have made prediction to 2037 (Figure 13 in http://globalclimatedrivers.blogspot.com ). Where can I find your prediction?
Ned (1:18 PM) – OK, I figured out what you are talking about regarding the significance of CO2. In my statement “The objective here …”, replace ‘has’ with ‘can have’ and add “Best estimate for the significance of CO2 using available data to date is shown in Table 1 row# M of http://globalclimatedrivers.blogspot.com . Figure 13 is the associated graph showing the anomaly comparisons.
DeleteNed (1:28 PM) – I have only done multiple linear regression analysis (decades ago) so I have no clue what tools are out there. I ran across this as a required condition for doing multiple regression “Assumption #6: Your data must not show multicollinearity, which occurs when you have two or more independent variables that are highly correlated with each other.” at https://statistics.laerd.com/spss-tutorials/multiple-regression-using-spss-statistics.php . It appears that the high correlation between CO2 and SSN would cause a problem.
TSI variation is way too small to directly account for AGT changes so an effect multiplier would need to be present. I don’t know of any for TSI but a possible one has been suggested with SSN as a proxy.
Ned (1:31 PM) & Nick – There is evidence it is not ‘crap’. I have been tracking the data changes. The growing separation between the reported surface data and reported satellite data is corroborative that the surface data has been jiggered.
A lot of paychecks depend on increased CO2 being a problem. It isn’t. For most of earth’s history CO2 level has been several times higher than the present. The planet plunged into the Andean-Saharan ice age when the CO2 level was over ten times higher than now. You can get links to this from my 2008 paper at http://www.middlebury.net/op-ed/pangburn.html
Nick (4:05 PM) –Multiply both sides of the equation by thcap to make it is easier to see that all factors are in terms of energy.
Ned (9:50 PM) – Thanks for the links. I use Law Dome for early, Moana Loa for recent and extrapolate using a 2nd order fit 1980-2012 for CO2 ppmv. I added a graph as Figure 4.5 to show this.
I had not seen the TSI source.
The influence coefficient for CO2 is an output of my analysis, not an input. I got a value of 0.188 compared to your 5.35.
It doesn’t work to do a different analysis, get a significantly different AGT from mine and claim mine is wrong. The only thing that is proven is that one of us is wrong. Right now AGT is corrupted by the on-going super El Nino. It will be revealing to see the AGT when its influence dies out. I have made prediction to 2037 (Figure 13 in http://globalclimatedrivers.blogspot.com ). Where can I find your prediction?
Interesting comment: I never saw until now such a pretty good CO2 conc/TSI comparison.
DeleteBut the question "Why mess around with temperature at all?" I can't quite follow.
In short papers published in 1901 and 1906, Arrhenius well confirmed that for him, the 'falsification' of his 1896 paper by Koch and Anders Angström's son Knut was bare nonsense.
But he was on the other hand also willing to accept a smaller amount of temp increase due to CO2.
I tried a little experiment
- using 50% of his ln constant, thus 2.68 instead of the original value;
- using as anomalies those of JMA's Tokio Climate center (a tick cooler than GISS/NOAA/BEST/HadCRUT4). Baseline here: 1981-2010.
The result: http://fs5.directupload.net/images/160519/gnecd8kk.jpg
"But the question 'Why mess around with temperature at all?' I can't quite follow."
DeleteObviously if you're trying to model climate change, then you need to somehow translate forcings into temperature changes.
But if you're just wanting to compare the relative importance of two different forcings, it's much easier to just do that comparison directly. Calculating the forcings associated with a change in CO2 and a change in TSI is straightforward. Then you have both in the same units (W m-2) without any need for the controversial stuff about how forcings translate into temperature changes.
Here's the result again. Very straightforward.
This doesn't tell us exactly how much temperature change will result from, say, increasing CO2 from 280 to 400 ppmv, but it does confirm that the historical range of solar variation has a much weaker effect on climate compared to that CO2 increase.
Dan said:
Delete"A lot of paychecks depend on increased CO2 being a problem. It isn’t. For most of earth’s history CO2 level has been several times higher than the present. The planet plunged into the Andean-Saharan ice age when the CO2 level was over ten times higher than now."
Motivated reasoning is the norm, for sure. Maybe the largest, most profitable, most influential global industrial complex has a few paychecks at risk, heh? Do you think the petroleum industry might be funding a little disinformation to downplay the influence of their CO2 emissions?
High CO2 in paleohistory is an old canard of the denial industry. I'm sure you are aware the the sun's output has increased about 7%/billion years. So the TSI during the Andean-Saharan ice age was about 3% lower. That is about 10 W/m^2 less energy from the sun. CO2 would have to be many times higher to maintain Earth's temperature. Did your motivated reasoning help you forget that fact?
Ned W: I of course agree to your position. DP's lack of logic brought me off line a bit :-)
DeleteBindidon:
DeleteThe formula used above for calculating radiative forcing from CO2 isn't Arrhenius's version. There have been several refinements over the years. Myhre et al. (1998; New estimates of radiative forcing due to well-mixed greenhouse gases) used line-by-line calculation of radiative transfer to determine the exact relationship between trace gas concentrations and radiative forcing. The equation used here (dF = 5.35 * ln[CO2_i/CO2_i0]) is a very close fit to Myhre's fully calculated version (according to a footnote in Myhre's Table 3, the 5.35 constant is accurate to within 1%).
In other words, it's an extremely close approximation to the actual relationship as derived from first principles. No GCMs or other "climate models" are used, just the fundamental radiative transfer equations. As far as I know, even "skeptics" like Lindzen, Spencer, and Christie all accept this derivation.
The difficult (or "controversial") part is how to translate this forcing (in W m-2) into a temperature change (in K).
By comparing radiative forcing from the sun, to radiative forcing from CO2, directly, in units of forcing, without worrying about temperatures, it's very straightforward to determine the relative importance of each factor.
I don't know how much warming CO2, or solar variation, has caused since 1700. But I do know that CO2 has caused 8.0 times as much warming as solar variation over that time period. That's easy to establish.
Ned (10:53 PM) – Temperature change is the time-integral of net forcing divided by effective thermal capacitance. That you appear to be unaware of this could be a problem.
DeleteWill (12:06 AM) – OK, think it through. The temperature dropped, big time, but then it came back up again to the level before it dropped. All at the high CO2 level. The observation that it came up again refutes that 3% lower TSI was a factor. A graph showing planet temperature and CO2 level over Geologic Time is at http://www.geocraft.com/WVFossils/Carboniferous_climate.html
Humanity has wasted over a trillion dollars in failed attempts using super computers to demonstrate that added atmospheric CO2 is a primary cause of global warming and in misguided activities to try to do something about it.
Ned (1:04 AM) – GW has to do with AGT. I have been unable to find how the 5.35 was arrived at or how to translate that assessment of CO2 influence into a meaningful AGT change. AGT is the relevant metric. Without a rational way to translate that assessment of CO2 forcing to calculated AGT and also have calculated AGT corroborated by measured AGT, it means nothing.
Meanwhile, the influence of CO2 forcing on AGT as determined by maximizing R^2 for Equation 1 is about 8% as documented in row #M of Table 1. This is a maximum based on AGT measurements to date. If future temperatures decline, as predicted (CO2 level will undoubtedly continue to increase), the calculated CO2 influence will decline from 8%.
DP: "the influence of CO2 forcing on AGT as determined by maximizing R^2 for Equation 1 is about 8% as documented in row #M of Table 1. This is a maximum based on AGT measurements to date."
DeleteHow can I say this politely enough for Nick's blog? You seriously misunderstand very basic concepts of statistics.
Your empirical (non-physical) model does not actually tell you anything about what fraction of warming is due to increasing CO2. Row "M" of your table does not in fact tell you that CO2 is only responsible for 8% of warming. The process you are using is quite literally incapable of providing the answer you think it provided.
Almost everything else you say is wrong, too. But this is probably the most egregious of your remarks.
Seriously, the problem is not my understanding of your (very simplified) cartoon model in equation 1. The problem is that you're using statistics to draw inferences that are wrong.
Apologies for the double-post.
ReplyDeleteNed,
DeleteThat's OK. You can delete your own comments at this site if you want - link is below comment. Default is that it leaves a marker (and the option to undelete). But there is a box you can tick which removes the comment totally (and finally).
Thanks, Nick. But I don't think that works when people post as Name/URL (as opposed to being logged in via Google or whatever). Otherwise, anyone could delete anyone else's comment.
DeleteWell, that might be interesting, but I don't think it's what Blogspot wants.
I'm always fascinated by the belief that when surface temperature undergo adjustments, it's considered scientific malpractice, but when satellite measurements undergo much larger changes, nary a peep is made. UAH can reduce the trend and go through 5 betas of ad hoc adjustments before even being submitted for peer review, but that's OK because Roy Spencer is good people. RSS was the golden boy to skeptics when it showed the least amount of warming, but only after it showed the most warming of any of the major analyses. Now that it shows more warming, it's back down the memory hole and TLT v4 hasn't even been released yet.
ReplyDeleteYes, CCE! And Nick's excellent plot comparing the differences between GISS revisions and those between UAH5.6 and 6.0beta is clear enough:
Deletehttps://i2.wp.com/www.moyhu.org.s3.amazonaws.com/2015/12/uahadj1.png
A comparison of the differences between the two UAH revisions a global level with their zonal differences at the poles gives the rest:
https://i1.wp.com/fs5.directupload.net/images/160508/eth49vwp.jpg
Roy Spencer never did feel any need to explain us how UAH's trend for the North Pole moved over night from 30% above RSS3.3 down to 30% below...
Moreover, a plot comparing between 1979 and 2016
- RSS3.3 TLT, UAH6.0beta5TLT, RSS4.0 TTT;
- RATPAC B monthly combined (surface, 500 hPa, 300 hPa);
- GISS
gives very interesting results:
- the TLT datasets coexist with RATPAC at 300 hPa (they should do at 700);
- RSS4.0 TTT and GISS TEMP(!!!) coexist with RATPAC at 500 hPa;
- RATPAC surf is far above all.
One might argue: well, that RATPAC has only 85 radiosondes, that's nothing reliable.
But
(1) John Christy compared himself his UAH product with radiosondes (but only till 2004, and based on TMT instead of TLT, hum hum);
(2) averaging, between 1979 and 2015, the data of all IGRA sondes (those the RATPAC sondes are a subset of) gives even much higher anomalies.
OOOps!
Deletefs5.directupload.net/images/160521/k66h3w98.pdf
cce, Roy Spencer adjusts every single satellite reading by use of a polynomial fit.
ReplyDeletehttp://www.drroyspencer.com/wp-content/uploads/AMSU5-gridpoint-polynomial-fit-example.gif
The adjustment range is more than 10 degrees Kelvin. Effectively this means that he adjust together readings from different heights in the atmosphere, that may differ more than 1km. I think the main purpose is to get more readings for each location during a month..
This is what Mr "I don't understand why people are doing adjustments" does :-)
Ned W on May 20, 2016 at 1:04 AM
ReplyDeleteThe formula used above for calculating radiative forcing from CO2 isn't Arrhenius's version.
You are right: I reread his 1896 publication, and there is no reference in it to such a formula. A. solely put in relation a geometric increase of CO2 concentration with an arithmetic increase of temperature.
The difficult (or "controversial") part is how to translate this forcing (in W m-2) into a temperature change (in K).
Indeed, and in fact I know that a relation between the two, like that insinuated in my comment on May 19, 2016 at 10:14 PM, is pure coincidence and has no scientific value at all. But amazing it is nevertheless :-)
But I do know that CO2 has caused 8.0 times as much warming as solar variation over that time period.
That's the lesson to retain I guess.
There's another non-physical term in the "balance" equation, inside the time integral:
ReplyDelete-F * [((T_i/T_1895)^4) - 1]
Where F can be 0 or 1.
Realistically, if you wanted to construct an energy balance, you might start with the yearly radiative flux:
j=A*T^4
In units W*yr*m^-2. Then, changes in flux relative to a baseline flux for 1895 (J_0) would come like this:
dj= J_i - J_0 = A* ( T_i^4 - T_0^4).
If you wanted to get dJ in relative terms, you could convert forms:
dJ = J_i - J_0
dJ = J_0 * (J_i/J_0 - 1)
dJ = J_0 * [(T_i/T_0)^4 - 1]
And you find that J_0 == F from Pangburn's equation. So to calculate the change in outgoing flux, you have to start with the initial outgoing flux.
To make the units fit, though, Pangburn flatly applies a value for the outgoing radiation: F = 1 W*yr*m^-2. Or sometimes F=0, implying that any energy the Earth gains, it keeps forever, which is non-physical.
Not that F = 1 makes any sense, either. If you wanted to define the outgoing flux as relative to a baseline of 1895, you'd need to start with a reasonable value for outgoing energy in 1895. But the outgoing radiation at TOA, the value for J_0 = F, would then be around 340 W/m^2, several orders of magnitude higher than Pangburn's number.
This means that radiating away accumulated energy from the Earth is about 340x harder in Pangburn's equation than in real life.
Y'know, for someone who rants so much about other people's ignorance of thermodynamics, it would be nice if Pangburn's equations were actually based in it. This is schlock that would get you a failing grade on an undergraduate physics/thermo project at any decent school in the country.
Pangburn, I'm sorry. No, it's not that we're biased, or that we like to circle the wagons. It's just that your equations are bad, and your math is unsound. Your work doesn't actually stand up.
DP's webpage is a fine example of fractal wrongness. You can pick any part of it, from the fundamental concept to the fine-scale details, and it'll turn out to be wrong. It's quite remarkable, actually.
DeleteThis might be trifling in the context of the above demolition jobs on DP's "contribution" to climate science but I was much bemused by the number of significant figures he quotes for R^2 i.e.
ReplyDelete"R2 was 0.972455, but continuing the convergence further to an indicated CO2 effectiveness of 8.9% only increased R2 to 0.974114."
Now there's a man who displays inordinate confidence in his abilities, a characteristic that seems to be pervasive among the denial community.