There is only a few hours left of May here, and the NCAR reanalysis has three days of data to go. May has had fewer extremes than earlier months, but warmed toward the end. It is currently showing the same average anomaly as February, and I think that is about where it will end up. That is actually not quite as warm as last May, or as March 2015, but it continues a warm start to the year. The GISS anomaly for February was 0.8°C.
Sunday, May 31, 2015
GWPF submission
Last month, I posted (here and here) about a GWPF call for submissions on temperature adjustments. I included a hasty draft, which people were kind enough to comment on. I hope I've incorporated most of those suggestions in this update. I have edited for sobriety and brevity, and shortened the blogpost inclusions. I have added a list of references. I have left the in-line links, because I doubt that many readers will have a paper-only copy; there is a list of urls at the end.
I'm planning to submit it within the next few days, unless readers think further improvement is required.
Update - I have sent it with the correction noted in comments.
I'm planning to submit it within the next few days, unless readers think further improvement is required.
Update - I have sent it with the correction noted in comments.
Friday, May 29, 2015
Daily Arctic Temperatures
People are watching the Arctic Sea Ice, which is melting rather fast this year. Often quoted is a plot of temperatures above 80°N from DMI. I'll show it below.
That plot is based on reanalysis data, and covers just one region. Since I am now regularly integrating current NCEP/NCAR reanalysis data (here), I thought I could do the same for that region, and the comparison would be a check. However, in some ways that high Arctic is not where melting is currently concentrated, so I thought I could also give a better spread of areas. Naturally, that means a gadget, below the fold.
The DMI plot shows an average from 1958-2002. That isn't without cost - it requires melding a number of different reanalyses to cover the range. It could be argued too that it misses recent Arctic warming, and so may show modern data as unusually warm, when by modern standards it isn't.
Anyway, I decided to stick with NCEP/NCAR data from 1994 to present, over which time it seems fairly reliable. And of course I use NCEP for current. There are some discrepancies. DMI aims to show the true surface temp 2m above the ground. I show what is basically the bottom grid level.
Here are the comparative plots for 2015. I've tried to stick to the DMI style here:
I have however used Celsius - I can't see the point of Kelvin here, especially with freezing being prominent. The plots are broadly similar. The DMI average has a longer period above zero, but to a smaller extent. This may reflect the slight difference in levels. DMI shows a warmer looking winter - reflecting I think the more ancient reference average.
So below the fold is the gadget. It lets you choose arbitrary rectangles on a 5×15° grid in the region above 60°N. I'll place it just below, with further description below that.
The "rectangle" shows with colored sides. Below is a box of controls. The colored triangles move the sides in the indicated directions by one step. Outward pointing triangles expand the area. The black triangles translate the rectangle by longitude. The initial rectangle has overlapping sides and doesn't show all the colors.
When you have selected the area, press "Plot new" to show the plot of daily average temperatures for 2015 to date. Day of year is marked on the x axis.
I'll add the plot to the maintained latest data page.
Update New post here. maintained location here.
That plot is based on reanalysis data, and covers just one region. Since I am now regularly integrating current NCEP/NCAR reanalysis data (here), I thought I could do the same for that region, and the comparison would be a check. However, in some ways that high Arctic is not where melting is currently concentrated, so I thought I could also give a better spread of areas. Naturally, that means a gadget, below the fold.
The DMI plot shows an average from 1958-2002. That isn't without cost - it requires melding a number of different reanalyses to cover the range. It could be argued too that it misses recent Arctic warming, and so may show modern data as unusually warm, when by modern standards it isn't.
Anyway, I decided to stick with NCEP/NCAR data from 1994 to present, over which time it seems fairly reliable. And of course I use NCEP for current. There are some discrepancies. DMI aims to show the true surface temp 2m above the ground. I show what is basically the bottom grid level.
Here are the comparative plots for 2015. I've tried to stick to the DMI style here:
DMI | NCEP/NCAR vai Moyhu |
![]() | ![]() |
I have however used Celsius - I can't see the point of Kelvin here, especially with freezing being prominent. The plots are broadly similar. The DMI average has a longer period above zero, but to a smaller extent. This may reflect the slight difference in levels. DMI shows a warmer looking winter - reflecting I think the more ancient reference average.
So below the fold is the gadget. It lets you choose arbitrary rectangles on a 5×15° grid in the region above 60°N. I'll place it just below, with further description below that.
The "rectangle" shows with colored sides. Below is a box of controls. The colored triangles move the sides in the indicated directions by one step. Outward pointing triangles expand the area. The black triangles translate the rectangle by longitude. The initial rectangle has overlapping sides and doesn't show all the colors.
When you have selected the area, press "Plot new" to show the plot of daily average temperatures for 2015 to date. Day of year is marked on the x axis.
I'll add the plot to the maintained latest data page.
Friday, May 22, 2015
How bad is naive temperature averaging?
In my last post, I described as "naive averaging" the idea of calculating an average temperature anomaly G by simply subtracting from each of a number of local records the (varying) lifetime average, and averaging the resulting differences. There, and in an earlier post I gave simple examples of why it didn't work. And in that last post, I showed how the naive average could be made right by iteration.
The underlying principle is that in making an anomaly you should subtract your best estimate of what the value would be. That leaves the question of how good does "best" have to be; it has to be good enough to resolve the thing you are trying to deduce. If that is the change of global temperature, your estimate has to be accurate to the effect of that change.
If you add a global G to a station mean, then the mean of the result isn't right unless mean G is zero. There is freedom to set average G over an interval, but only one - not over all station intervals. So as in the standard method, you can set G to have mean zero over a period like 1961-90, and use station means over that period as the offsets. Providing there are observations there, which is the rub. But much work has been done on methods for this, which itself is evidence that the faults of naive averaging are well known.
Anyway, here I want to check just how much difference the real variation of intervals in temperature datasets makes, and whether the iterations (and TempLS) do correct it properly. I take my usual collection of GHCN V3 and ERSST data, at 9875 locations total, and the associated area-based weights, which are zero for all missing values. But then I change the data to a uniformly rising value - in fact, equal to the time in century units since the start in 1899. That implies a uniform trend of 1 C/century. Do the various methods recover that?
Update - I made a small error in trends below. I used unweighted regressions, which allowed the final months of 2015 to be entered as zeroes. I have fixed this, and put the old tables in faint gray beside. The plots were unaffected. I have not corrected the small error I noted in the comments, but now it is even smaller - a converged trend of 0.9998 instead of 1.
So I'll start with land only (GHCN) data - 7280 stations - because most of the naive averaging is done with land stations. Here is the iterative sequence:
As in the last post, the first iteration is the naive calculation, where the offsets are just the means over the record length. RMS is a normalised sqrt sum squares of residuals. As you see, the trend for that first step was about half the final value. And it does converge to 1C/Cen, as it should. This is the value TempLS would return.
I should mention that I normalised the global result at each step relative to the year 2014. Normally this can be left to the end, and would be done for a range of years. Because of the uniformity of the data, setting to a one year anomaly base is adequate. For annual data, base setting is just the addition of a constant. For monthly data, there are twelve different constants, so the anomaly base setting has the real effect of adjusting months relative to each other. It makes a small difference within each year, but if not set till the end, the convergence of the trend to 1 is not so evident, though the final result is the same.
So normalised to 2014, here is the plot of the global temperatures at each iteration:
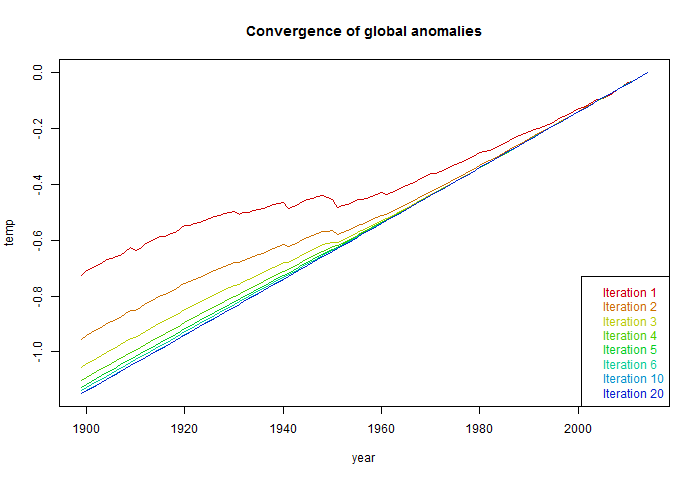
The first naive iteration deviates quite substantially, with reduced trend. The deviation is due solely to the pattern of missing values.
Now I'll do the same calculation including sea surface temperature - the normal TempLS range. The effects are subdued, because SST grid values don't generally have start and end years like met stations, even though they may have missing values.
The corresponding plot of iterative G curves is:
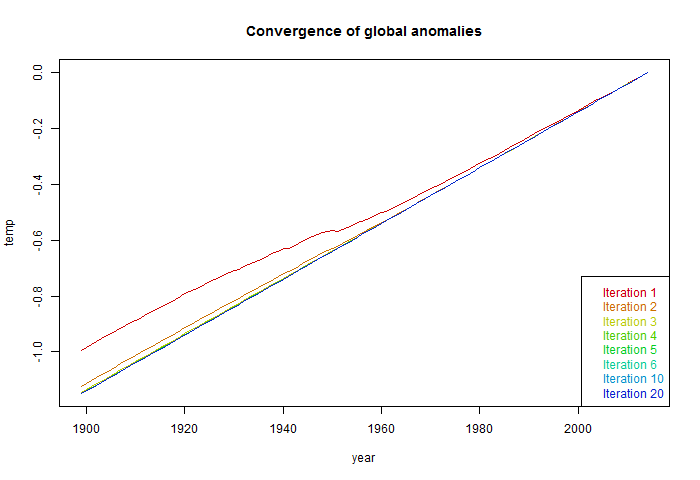
The deviation of the first step is reduced, but still considerable. Convergence is faster.
The underlying principle is that in making an anomaly you should subtract your best estimate of what the value would be. That leaves the question of how good does "best" have to be; it has to be good enough to resolve the thing you are trying to deduce. If that is the change of global temperature, your estimate has to be accurate to the effect of that change.
If you add a global G to a station mean, then the mean of the result isn't right unless mean G is zero. There is freedom to set average G over an interval, but only one - not over all station intervals. So as in the standard method, you can set G to have mean zero over a period like 1961-90, and use station means over that period as the offsets. Providing there are observations there, which is the rub. But much work has been done on methods for this, which itself is evidence that the faults of naive averaging are well known.
Anyway, here I want to check just how much difference the real variation of intervals in temperature datasets makes, and whether the iterations (and TempLS) do correct it properly. I take my usual collection of GHCN V3 and ERSST data, at 9875 locations total, and the associated area-based weights, which are zero for all missing values. But then I change the data to a uniformly rising value - in fact, equal to the time in century units since the start in 1899. That implies a uniform trend of 1 C/century. Do the various methods recover that?
Update - I made a small error in trends below. I used unweighted regressions, which allowed the final months of 2015 to be entered as zeroes. I have fixed this, and put the old tables in faint gray beside. The plots were unaffected. I have not corrected the small error I noted in the comments, but now it is even smaller - a converged trend of 0.9998 instead of 1.
So I'll start with land only (GHCN) data - 7280 stations - because most of the naive averaging is done with land stations. Here is the iterative sequence:
|
|
As in the last post, the first iteration is the naive calculation, where the offsets are just the means over the record length. RMS is a normalised sqrt sum squares of residuals. As you see, the trend for that first step was about half the final value. And it does converge to 1C/Cen, as it should. This is the value TempLS would return.
I should mention that I normalised the global result at each step relative to the year 2014. Normally this can be left to the end, and would be done for a range of years. Because of the uniformity of the data, setting to a one year anomaly base is adequate. For annual data, base setting is just the addition of a constant. For monthly data, there are twelve different constants, so the anomaly base setting has the real effect of adjusting months relative to each other. It makes a small difference within each year, but if not set till the end, the convergence of the trend to 1 is not so evident, though the final result is the same.
So normalised to 2014, here is the plot of the global temperatures at each iteration:
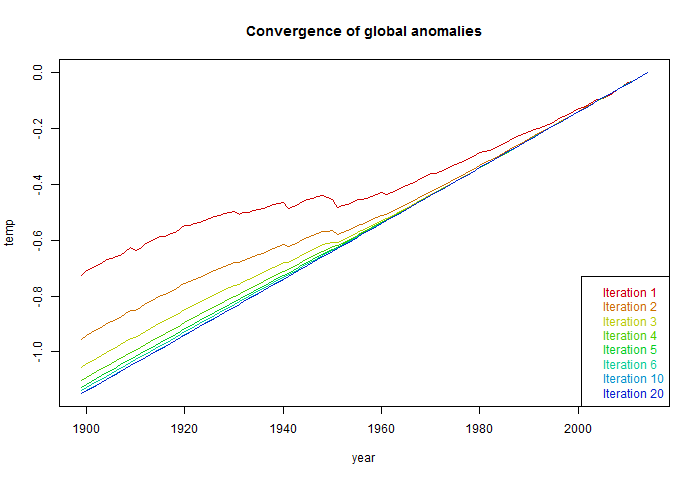
The first naive iteration deviates quite substantially, with reduced trend. The deviation is due solely to the pattern of missing values.
Now I'll do the same calculation including sea surface temperature - the normal TempLS range. The effects are subdued, because SST grid values don't generally have start and end years like met stations, even though they may have missing values.
|
|
The corresponding plot of iterative G curves is:
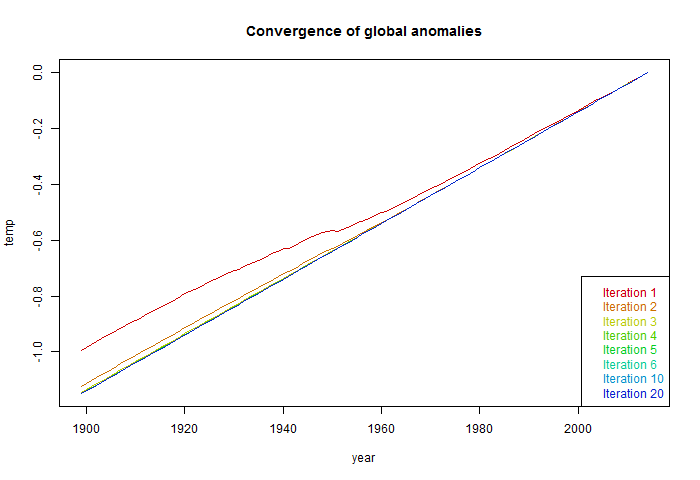
The deviation of the first step is reduced, but still considerable. Convergence is faster.
Monday, May 18, 2015
How to average temperature over space and time
This post is about the basics of TempLS. But I want to present it in terms of first principles of averaging, with reference to other methods that people try that just don't work. I'll mention the least squares approach described here.
I once described a very simple version of TempLS. In a way, what I am talking about here is even simpler. It incorporates an iterative approach which not only gives context to the more naive approaches, which are in fact the first steps, but is a highly efficient solution process.
I'm going to average this over time and space. It will be a weighted average, with a weight array wsmy exactly like x. The first thing about w is that it has a zero entry wherever data is missing. Then the result is not affected by the value of x, which I usually set to 0 there for easier linear algebra in R.
The weighting will generally be equal over time. The idea is that averaging for a continuum (time or space) generally means integration. Time steps are equally spaced.
Spatial integration is more complicated. The basic idea is to divide the space into small pieces, and estimate each piece by local data. In effect, major indices do this by gridding. The estimate for each cell is the average of datapoints within, for a particular time. The grid is usually lat/lon, so the trig formula for area is needed. The big problem is cells with no data. Major indices use something equivalent to this.
Recently I've been using a finite element style method - based on triangular meshing. The surface is completely covered with triangles with a datapoint at each corner (picture here). The integral is that of the linear interpolation within each triangle. This is actually higher order accuracy - the 2D version of the trapezoidal method. The nett result is that the weighting of each datapoint is proportional to the area of the triangles of which it is a vertex (picture here).
The key issue is missing values. There is one type of familiar missing value - where a station does not report in some month. As an extension, you can think of the times before it started or after it closed as missing values.
But in fact all points on Earth except the sample points can be considered missing. I've described how the averaging deals with them. It interpolates and then integrates that. So the issue with averaging is whether that interpolation is good.
The answer for absolute temperatures is,no it isn't. They vary locally within any length scale that you can possibly sample. And anyway, we get little choice about sampling.
I've written a lot about where averaging goes wrong, as it often does. The key thing to remember is that if you don't prescribe infilling yourself, something else will happen by default. Usually it is equivalent to assigning to missing points the average value of the remainder. This may be very wrong.
A neat way of avoiding most difficulties is the use of anomalies. Here you subtract your best estimate of the data, based on prior knowledge. The difference, anomaly, then has zero expected value. You can average anomalies freely because the default, the average of the remainder, will also be zero. But it should be your best estimate - if not, then there will be a systematic deviation which can bias the average when missing data is defaulted. I'll come back to that.
xsmy=Lsm+Gmy+εsmy
Here L is a local offset for station month (like a climate normal), G is a global temperature function and ε an error term. Suffices show what they depend on.
TempLS minimises the weighted sum of squares of residuals:
Σwsmydsmy² where dsmy=xsmy-Lsm-Gmy
It is shown here that leads to two equations in L and G which TempLS solves simultaneously. But here I want to give them an intuitive explanation. The local offsets don't vary with year. So you can get an estimate by averaging the model over years. That gives an equation for each L.
And the G parameters don't involve the station s. So you can estimate those by averaging over stations. That would be a weighted average with w.
Of course it isn't as simple as that. Averaging over time to get L involves the unknown G. But it does mean, at least, that we have one equation for each unknown. And it does allow iteration, where we successively work out L and G, at each step using the latest information available. This is a well-known iterative method - Gauss-Seidel.
That's the approach I'll be describing here. It works well, but also is instructive. TempLS currently uses either block Gaussian elimination with a final direct solve of a ny×ny matrix (ny=number years), or conjugate gradients, which is an accelerated version of the iterative method.
But one that is common, and sometimes not too bad, is where a normal is first calculated for each station/month over the period of data available, and used to create anomalies that are averaged. I gave a detailed example of how that goes wrong here. Hu McCulloch gave one here.
The basic problem is this. In setting T=L+G as a model, there is an ambiguity. L is fixed over years, G over space. But you could add any number to the L's as long as you subtract it from G. If you want L to be the mean over an interval, you'll want G to have zero mean. But If stations have a variety of intervals, G can't be zero-mean over all of them.
The normal remedy is to adopt a fixed interval, say 1961-90, on which G is constrained to have zero mean. Then the L's will indeed be the mean for each station for that interval, if they have one. The problem of what to do if not has been much studied. There's a discussion here.
Σw_y(d_smy) = 0
or Σw_y(L_sm) = Σw_y(T_smy - G_my)......Eq(1)
But Σw_y(L_sm) = L_sm Σw_y(1). Eq(1) is solvable for L_sm
and
Σw_s(d_smy) = 0
or Σw_s(G_my) = Σw_y(T_smy - L_sm)......Eq(2)
But Σw_s(G_my) = G_my Σw_s(1). Eq(2) is solvable for G_my
The naive model is in fact a first step. Start with G=0. Solve (1) gives the normals, and solve (2) gives the resulting anomaly average.
It's not right, because as said, with non-zero G, (1) is not satisfied. But you can solve again, with the new G. Then solve (2) again with the new L. Repeat. When (and if) it has converged, both equations are satisfied.
and w looks like
Here are the first four iterations. I've calculated the trend of G, which should be 1, and the weighted sum of squares of residuals.
The first row is the naive recon. The trend is way too low, and the sum squares is far from zero. Iteration shows gradual improvement. The columns do converge to 1 and 0 respectively.
The convergence speed depends on the overlap between stations. If there had not been overlap in the middle, the algorithm would have happily reported a zig-zag with the anomaly of each station as the global value. If I had extended the range of each station by 1 year, convergence is rapid. A real example would have lots of overlap. Here are plots of the comvergence:
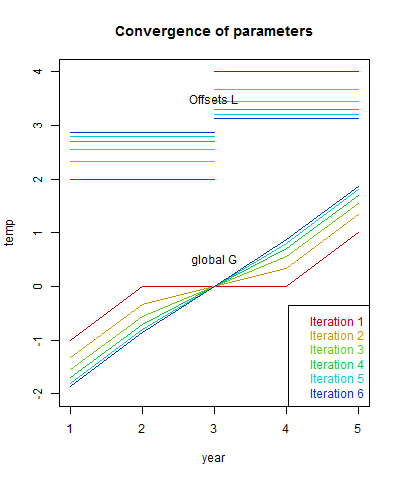
Again, the first row, corresponding to the naive method, significantly understates the whole-period trend. The sum of squares does not change much, because most of the monthly deviation is not explained by global change. The weights I used were mesh-based, and as a quick check with the regular results
To 3 figures, it is the same.
I once described a very simple version of TempLS. In a way, what I am talking about here is even simpler. It incorporates an iterative approach which not only gives context to the more naive approaches, which are in fact the first steps, but is a highly efficient solution process.
Preliminaries - averaging methods
The first thing done in TempLS is to take a collection of monthly station readings, not gridded, and arrange them in a big array by station, month and year. I denote this xsmy. Missing values are marked with NA entries. Time slots are consecutive. "Station" can mean a grid cell for SST, assigned to the central location.I'm going to average this over time and space. It will be a weighted average, with a weight array wsmy exactly like x. The first thing about w is that it has a zero entry wherever data is missing. Then the result is not affected by the value of x, which I usually set to 0 there for easier linear algebra in R.
The weighting will generally be equal over time. The idea is that averaging for a continuum (time or space) generally means integration. Time steps are equally spaced.
Spatial integration is more complicated. The basic idea is to divide the space into small pieces, and estimate each piece by local data. In effect, major indices do this by gridding. The estimate for each cell is the average of datapoints within, for a particular time. The grid is usually lat/lon, so the trig formula for area is needed. The big problem is cells with no data. Major indices use something equivalent to this.
Recently I've been using a finite element style method - based on triangular meshing. The surface is completely covered with triangles with a datapoint at each corner (picture here). The integral is that of the linear interpolation within each triangle. This is actually higher order accuracy - the 2D version of the trapezoidal method. The nett result is that the weighting of each datapoint is proportional to the area of the triangles of which it is a vertex (picture here).
Averaging principles
I've described the mechanics of averaging (integrating) over a continuum. Now it's time to think about when it will work. We are actually estimating by sampling, and the samples can be sparse.The key issue is missing values. There is one type of familiar missing value - where a station does not report in some month. As an extension, you can think of the times before it started or after it closed as missing values.
But in fact all points on Earth except the sample points can be considered missing. I've described how the averaging deals with them. It interpolates and then integrates that. So the issue with averaging is whether that interpolation is good.
The answer for absolute temperatures is,no it isn't. They vary locally within any length scale that you can possibly sample. And anyway, we get little choice about sampling.
I've written a lot about where averaging goes wrong, as it often does. The key thing to remember is that if you don't prescribe infilling yourself, something else will happen by default. Usually it is equivalent to assigning to missing points the average value of the remainder. This may be very wrong.
A neat way of avoiding most difficulties is the use of anomalies. Here you subtract your best estimate of the data, based on prior knowledge. The difference, anomaly, then has zero expected value. You can average anomalies freely because the default, the average of the remainder, will also be zero. But it should be your best estimate - if not, then there will be a systematic deviation which can bias the average when missing data is defaulted. I'll come back to that.
What TempLS does
I'll take up the story where we have a data array x and weights w. As described here, TempLS represents the data by a linear modelxsmy=Lsm+Gmy+εsmy
Here L is a local offset for station month (like a climate normal), G is a global temperature function and ε an error term. Suffices show what they depend on.
TempLS minimises the weighted sum of squares of residuals:
Σwsmydsmy² where dsmy=xsmy-Lsm-Gmy
It is shown here that leads to two equations in L and G which TempLS solves simultaneously. But here I want to give them an intuitive explanation. The local offsets don't vary with year. So you can get an estimate by averaging the model over years. That gives an equation for each L.
And the G parameters don't involve the station s. So you can estimate those by averaging over stations. That would be a weighted average with w.
Of course it isn't as simple as that. Averaging over time to get L involves the unknown G. But it does mean, at least, that we have one equation for each unknown. And it does allow iteration, where we successively work out L and G, at each step using the latest information available. This is a well-known iterative method - Gauss-Seidel.
That's the approach I'll be describing here. It works well, but also is instructive. TempLS currently uses either block Gaussian elimination with a final direct solve of a ny×ny matrix (ny=number years), or conjugate gradients, which is an accelerated version of the iterative method.
Naive methods
A naive method that I have inveighed against is to simply average the absolute temperatures. That is so naive that it is, of course, never done with proper weighting. But weighting isn't the problem.But one that is common, and sometimes not too bad, is where a normal is first calculated for each station/month over the period of data available, and used to create anomalies that are averaged. I gave a detailed example of how that goes wrong here. Hu McCulloch gave one here.
The basic problem is this. In setting T=L+G as a model, there is an ambiguity. L is fixed over years, G over space. But you could add any number to the L's as long as you subtract it from G. If you want L to be the mean over an interval, you'll want G to have zero mean. But If stations have a variety of intervals, G can't be zero-mean over all of them.
The normal remedy is to adopt a fixed interval, say 1961-90, on which G is constrained to have zero mean. Then the L's will indeed be the mean for each station for that interval, if they have one. The problem of what to do if not has been much studied. There's a discussion here.
Iterating
I'll introduce a notation Σw_s, Σw_y, meaning that a w-weighted sum is formed over the suffix variable (station, year) holding the others fixed. I'd call it an average, but the weights might not add to 1. So Σw_y yields an array with values for each station and month. Our equations are thenΣw_y(d_smy) = 0
or Σw_y(L_sm) = Σw_y(T_smy - G_my)......Eq(1)
But Σw_y(L_sm) = L_sm Σw_y(1). Eq(1) is solvable for L_sm
and
Σw_s(d_smy) = 0
or Σw_s(G_my) = Σw_y(T_smy - L_sm)......Eq(2)
But Σw_s(G_my) = G_my Σw_s(1). Eq(2) is solvable for G_my
The naive model is in fact a first step. Start with G=0. Solve (1) gives the normals, and solve (2) gives the resulting anomaly average.
It's not right, because as said, with non-zero G, (1) is not satisfied. But you can solve again, with the new G. Then solve (2) again with the new L. Repeat. When (and if) it has converged, both equations are satisfied.
Code
Here is some R code that I use. It starts with x and w arrays (dimensioned ns×nm×ny×, nm=12,nsm=ns*nm) as defined above:G=0; wx=w*x; wr=rowSums(w)+1e-9; wc=colSums(w);
for(i in 1:8){
L=rowSums(wx-w*rep(G,each=nsm))/wr
G=colSums(wx-w*rep(L,ny))/wc
}
That's it. The rowSums etc do the averaging. I add 1e-9 to wr in case stations have no data for a given month. That would produce 0/0, which I want to be 0. I should mention that I have set all the NAs in x to 0. That's OK, because they have zero weight. Of course, in practice I monitor convergence and end the loop when achieved. We'll see that later.
In words
- Initially, the offsets L are the long term station monthly averages
- Anomalies are formed by subtracting L, and the global G by spatially averaging (carefully) the anomalies. Thus far, the naive method.
- Form new offsets by subtracting the global anomaly from measured T, and averaging that over years.
- Use the new offsets to make a spatially averaged global anomaly
- Repeat 3 and 4 till converged.
A simple example
Here's another simple example showing that just taking anomalies over station data isn't enough. We'll forget months; the climate is warming 1C/year (change the units if you like), and there are two stations which measure this exactly over five years. But they have missing readings. So the x array looks like this:Station A | 1 | 2 | 3 | - | - |
Station B | - | - | 3 | 4 | 5 |
w | 1 | 1 | 1 | 0 | 0 |
0 | 0 | 1 | 1 | 1 |
j | ||
Iter | Trend | Sum squares |
1 | 0.4 | 2 |
2 | 0.6 | 0.8889 |
3 | 0.7333 | 0.3951 |
4 | 0.8222 | 0.1756 |
The convergence speed depends on the overlap between stations. If there had not been overlap in the middle, the algorithm would have happily reported a zig-zag with the anomaly of each station as the global value. If I had extended the range of each station by 1 year, convergence is rapid. A real example would have lots of overlap. Here are plots of the comvergence:
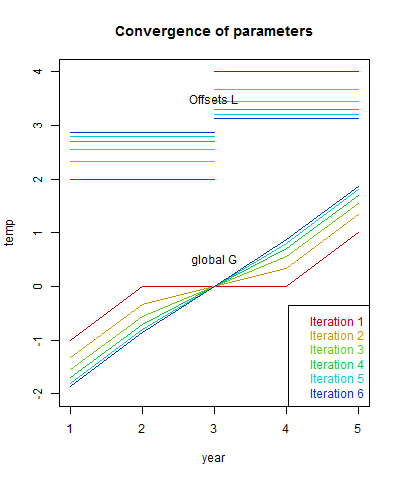
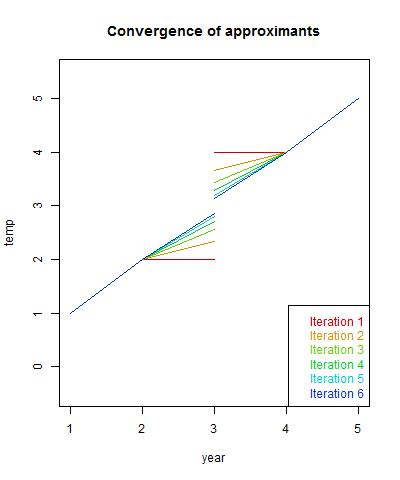
A real example
Now I'll take x and w from a real TempLS run, from 1899 to April 2015. They are 9875×12×117 arrays, and returns 12*117 monthly global anomalies G. I'll give the complete code below. It takes four seconds on my PC to do six iterations:Iter | Trend | Sum squares |
1 | 0.5921 | 1 |
2 | 0.6836 | 0.994423 |
3 | 0.6996 | 0.994266 |
4 | 0.7026 | 0.99426 |
5 | 0.7032 | 0.99426 |
6 | 0.7033 | 0.99426 |
Again, the first row, corresponding to the naive method, significantly understates the whole-period trend. The sum of squares does not change much, because most of the monthly deviation is not explained by global change. The weights I used were mesh-based, and as a quick check with the regular results
Nov | Dec | Jan | Feb | Mar | Apr | |
Regular run 18 May | 0.578 | 0.661 | 0.662 | 0.697 | 0.721 | 0.63 |
This post | 0.578 | 0.661 | 0.662 | 0.697 | 0.721 | 0.630 |
More code
So here is the R code including preprocessing, calculation and diagnostics. I've used color to show these various parts:for(i in 1:8){ load("TempLSxw.sav") # x are raw temperatures, w wts from TempLS x[is.na(x)]=0 d=dim(x); # 9875 12 117 L=v=0; wr=rowSums(w,dims=2)+1e-9; # right hand side wc=colSums(w)+1e-9; # right hand side for(i in 1:6){ L=rowSums(w*x-w*rep(G,each=d[1]),dims=2)/wr # Solve for L G=colSums(w*x-w*rep(L,d[3]))/wc # then G r=x-rep(L,ny)-rep(G,each=ns) # the residuals v=rbind(v,round(c(i, lm(c(G)~I(1:length(G)))$coef[2]*1200,sum(r*w*r)),4)) } colnames(v)=c("Iter","Trend","Sum sq re w") v[,3]=round(v[,3]/v[2,3],6) # normalising SS G=G-rowMeans(G[,62:91+1]) # to 1961-90 anomaly base write(html.table(v),file="tmp.txt")
Sunday, May 17, 2015
Emissions hiatus?
John Baez has a post on the latest preliminary data from EIA for 2014. Global CO2 emissions are the same as for 2013 at 32.3 Gtons. EIA says that it is the first non-increase for 40 years that was not tied to an economic downturn.
They attribute the pause to greater use of renewables, mentioning China. Greenpeace expands on this, saying that China's use of coal dropped by 8%, with a consequent 5% drop in CO2 emission. They give the calc with sources here. This source says April coal mined in China was down 7.4% on last year, which they do partly attribute to economic slowdown there.
It's just one year, and may be influenced by China's economy. We'll see.
They attribute the pause to greater use of renewables, mentioning China. Greenpeace expands on this, saying that China's use of coal dropped by 8%, with a consequent 5% drop in CO2 emission. They give the calc with sources here. This source says April coal mined in China was down 7.4% on last year, which they do partly attribute to economic slowdown there.
It's just one year, and may be influenced by China's economy. We'll see.
Friday, May 15, 2015
JAXA Hiatus
If you're following JAXA Arctic sea ice, they have announced an interruption for maintenance, until May 20. Pity, it is getting interesting, with 2015 now at record low levels for the day. Maybe it was going off the rails - we'll see.
NSIDC NH isn't looking so reliable either - they showed a massive re-freeze yesterday.
Update - JAXA has unhelpfully taken down all its data, and replaced it with the warning (in Japanese), which overwrote my local data. So no JAXA table data here till that is restored. Fortunately the plot is OK.
Update - Jaxa is back.
NSIDC NH isn't looking so reliable either - they showed a massive re-freeze yesterday.
Update - JAXA has unhelpfully taken down all its data, and replaced it with the warning (in Japanese), which overwrote my local data. So no JAXA table data here till that is restored. Fortunately the plot is OK.
Update - Jaxa is back.
Thursday, May 14, 2015
GISS down by 0.1°C in April
GISS has reported an average anomaly of 0.75°C (h/t JCH). They raised their March estimate to 0.85°C (from 0.84), so that makes a difference of 0.1, which is what I also reported for TempLS. In fact, TempLS has since crept up with extra data, so the difference is 0.09°C. Metzomagic comments on recent GISS changes here.
Update: GISS has published an update
Below the fold, as usual, I'll show the GISS plot, and the TempLS spherical harmonics smoothed map.
Here is the GISS map
And here is the TempLS spherical harmonics smoothed map:
And here is a listing of many corresponding TempLS monthly reports in recent years.
Update: GISS has published an update
May 15, 2015: Due to an oversight several Antarctic stations were excluded from the analysis on May 13, 2015. The analysis was repeated today after including those stations.This actually made a big difference. The April temperature anomaly comes back from 0.75°C to 0.71°C, and March from 0.85°C to 0.84, making a drop of 0.13°C. GISS uses an extra Antarctic data set from GHCN, which I presume is the one involved. So this won't affect the TempLs calc.
Below the fold, as usual, I'll show the GISS plot, and the TempLS spherical harmonics smoothed map.
Here is the GISS map
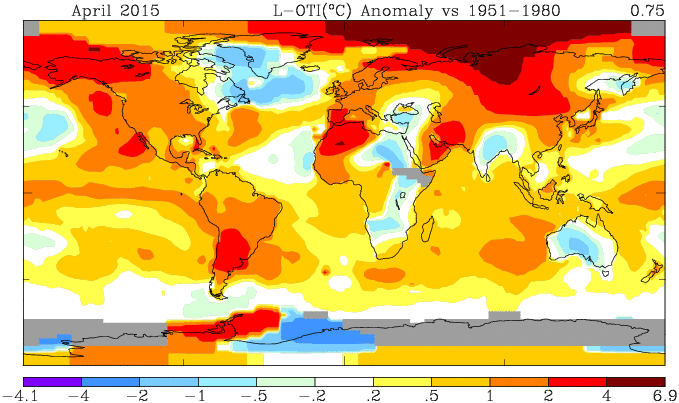
And here is the TempLS spherical harmonics smoothed map:
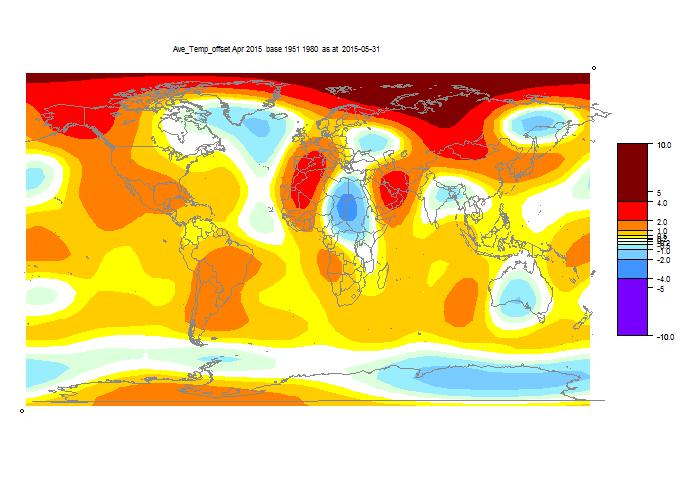
And here is a listing of many corresponding TempLS monthly reports in recent years.
Tuesday, May 12, 2015
BoM declares El Niño status
Australia's Bureau of Meteorology has upgraded its ENSO Tracker to El Niño status, saying
Sou has more on the story here.
ps In terms of Arctic sea ice, 2015 has been lately well ahead in melting of most recent years. It has just passed 2006 (which is fading after an early spurt) to have the least ice for this day (12 May).
"El Niño–Southern Oscillation (ENSO) indicators have shown a steady trend towards El Niño levels since the start of the year. Sea surface temperatures in the tropical Pacific Ocean have exceeded El Niño thresholds for the past month, supported by warmer-than-average waters below the surface. Trade winds have remained consistently weaker than average since the start of the year, cloudiness at the Date Line has increased and the Southern Oscillation Index (SOI) has remained negative for several months. These indicators suggest the tropical Pacific Ocean and atmosphere have started to couple and reinforce each other, indicating El Niño is likely to persist in the coming months.You can see an animation of the last 50 days of ENSO-responsive SST here.
International climate models surveyed by the Bureau indicate that tropical Pacific Ocean temperatures are likely to remain above El Niño thresholds through the coming southern winter and at least into spring."
Sou has more on the story here.
ps In terms of Arctic sea ice, 2015 has been lately well ahead in melting of most recent years. It has just passed 2006 (which is fading after an early spurt) to have the least ice for this day (12 May).
Monday, May 11, 2015
Friday, May 8, 2015
TempLS April cooler by 0.1°C
As foreshadowed from the daily NCEP reanalysis, April surface temperatures were down, according to TempLS, from 0.721°C to 0.623°C (as at 8/5). Data came in early, though I don't think we have all of Canada. Warm in central Russia, West N America, an odd pattern in Africa, which may change with more data. I'll just paste the report below.
Update. I notice Roy Spencer's map has the same major features as the one below.
Update. I notice Roy Spencer's map has the same major features as the one below.
Wednesday, May 6, 2015
New Page - Google Maps and GHCN
I've created a new page which combines some of the Google maps capability that I have been developing. I'll include the full text below, but the post is showing on the right; also it is here.
This page shows in a Google maps framework the 7280 stations of the GHCN Monthly network. To the side is a panel where you can choose which stations will be shown, and with what colors. You can select by metadata like altitude or urban status, or by ranges of data. here the data is a variety of trend options over the last 30, 50 or 60 years. The options are trends from the GHCN unadjusted data file, or adjusted, or the difference if you want to see what effect adjustment had.
I'll explain methods below the gadget.
The initial display shows an arbitrary selection. It has available all the usual Google Maps facilities, with controls at top left. But particularly you can click on any marker to bring up a balloon of information. This includes name, a link to the NOAA data sheet attached to the GHCN number, some metadata, and a trend number.
At the bottom of the box on the right, there is a selection box which determines that trend type. It has combinations of Unadj, Adj and A-U, meaning GHCN trend of unadjusted, adjusted and the trend difference. Choosing there determines what is meant by "Trend" elsewhere.
The green box on the right has a collection of selection criteria. Some are comparisons, some are logical. The second small button toggles between the relation options (>,==,T/F etc), and for comparison, the third is a text box in which you enter the reference value. Only one selection can be live at a time, determined by the left radio button. If nothing is live, nothing happens.
When you have a live selection, you can click a radio button in the top orange section (Pink,Cyan etc). Any stations that fulfill your requirement will change to that color. In the middle column, the numbers in each color are shown, and updated with each choice. Invisibles are still in the totals. This is a very handy way of displaying a count of a subset.
The right column shows the most recent logical operation that was implemented for that color. It's just a reminder and does not show the status of all markers in that color. If the color is eg pink, then the expression will not include markers that were pink before the latest selection, and the other logicals don't change.
The All button, when F does nothing, but when T and live changes everything to one color. You may want to start with everything invisible. I'd make this the default, except that it is a bit discouraging when the screen first comes up.
You can enter NaN into the text fields, which will have the effect of changing any NaN to that color. Usually used to make them invisible. For Trend_Adj, there is the option of equality ("=="), mainly used to test for zero. I should warn that it tests to rounding level, which is 0.01. Very few stations totally escape adjustment (eg MMTS), but it is often very small.
I've included Lat and Lon; it doesn't mean much when you have a map. but is useful for counting, eg Arctic. I have given Urban and Rural as separate options, because there is also Mixed. So if you color Urban T, that is what you see, but Rural F gives Urban and Mixed.
You'll find negative logic useful. The advice on how to sculpt an elephant is, take a very big rock and chip away anything that doesn't look like an elephant. Same here. If you want pink to show urban stations that have trend increased on adjustment, then pink all uptrended, then go to Urban F, and make that invisible. That will affect other colors too (if any).
Earlier Google maps posts include:
This page shows in a Google maps framework the 7280 stations of the GHCN Monthly network. To the side is a panel where you can choose which stations will be shown, and with what colors. You can select by metadata like altitude or urban status, or by ranges of data. here the data is a variety of trend options over the last 30, 50 or 60 years. The options are trends from the GHCN unadjusted data file, or adjusted, or the difference if you want to see what effect adjustment had.
I'll explain methods below the gadget.
The initial display shows an arbitrary selection. It has available all the usual Google Maps facilities, with controls at top left. But particularly you can click on any marker to bring up a balloon of information. This includes name, a link to the NOAA data sheet attached to the GHCN number, some metadata, and a trend number.
At the bottom of the box on the right, there is a selection box which determines that trend type. It has combinations of Unadj, Adj and A-U, meaning GHCN trend of unadjusted, adjusted and the trend difference. Choosing there determines what is meant by "Trend" elsewhere.
The green box on the right has a collection of selection criteria. Some are comparisons, some are logical. The second small button toggles between the relation options (>,==,T/F etc), and for comparison, the third is a text box in which you enter the reference value. Only one selection can be live at a time, determined by the left radio button. If nothing is live, nothing happens.
When you have a live selection, you can click a radio button in the top orange section (Pink,Cyan etc). Any stations that fulfill your requirement will change to that color. In the middle column, the numbers in each color are shown, and updated with each choice. Invisibles are still in the totals. This is a very handy way of displaying a count of a subset.
The right column shows the most recent logical operation that was implemented for that color. It's just a reminder and does not show the status of all markers in that color. If the color is eg pink, then the expression will not include markers that were pink before the latest selection, and the other logicals don't change.
The All button, when F does nothing, but when T and live changes everything to one color. You may want to start with everything invisible. I'd make this the default, except that it is a bit discouraging when the screen first comes up.
You can enter NaN into the text fields, which will have the effect of changing any NaN to that color. Usually used to make them invisible. For Trend_Adj, there is the option of equality ("=="), mainly used to test for zero. I should warn that it tests to rounding level, which is 0.01. Very few stations totally escape adjustment (eg MMTS), but it is often very small.
I've included Lat and Lon; it doesn't mean much when you have a map. but is useful for counting, eg Arctic. I have given Urban and Rural as separate options, because there is also Mixed. So if you color Urban T, that is what you see, but Rural F gives Urban and Mixed.
You'll find negative logic useful. The advice on how to sculpt an elephant is, take a very big rock and chip away anything that doesn't look like an elephant. Same here. If you want pink to show urban stations that have trend increased on adjustment, then pink all uptrended, then go to Urban F, and make that invisible. That will affect other colors too (if any).
Earlier Google maps posts include:
- Google Maps and GHCN adjustments
- Google Maps App showing GHCN adjustments.
- Google Maps portal to NOAA station histories.
- Universal station locator and history plotter
- New ISTI Temperature database - stations in Google Maps ...
- Google Maps display of GHCN stations, with ...
- Google Map problem fixed
Saturday, May 2, 2015
An early GHCN V1 found - hardly different from latest V3 unadjusted
Clive Best has come up with an early version of GHCN V1, which he says is from around 1990; GHCN V1 was released in 1992, and I think this must be from a late stage of preparation. Anyway, he has kindly made it available. I have been contending that GHCN unadjusted is essentially unchanged , except by addition, since the inception, despite constant loud claims that NOAA is constantly altering history.
I thought I would compare with the latest GHCN V3 unadjusted, which has data up to March 2015. This turns out to be not so simple, as the numbering schemes for stations have changed slightly, as have the recorded station names. Stations have a WMO number, but several can share the same number in both sets. They have a modification number, but these don't match.
So for this post, I settled on just one country, Iceland. GHCN is supposed to have done terrible things to this record. A few weeks ago, we had Chris Booker in the Telegraph:
"When future generations look back on the global-warming scare of the past 30 years, nothing will shock them more than the extent to which the official temperature records - on which the entire panic ultimately rested - were systematically "adjusted" to show the Earth as having warmed much more than the actual data justified.
...
Again, in nearly every case, the same one-way adjustments have been made, to show warming up to 1 degree C or more higher than was indicated by the data that was actually recorded. This has surprised no one more than Traust Jonsson, who was long in charge of climate research for the Iceland met office (and with whom Homewood has been in touch). Jonsson was amazed to see how the new version completely "disappears" Iceland's "sea ice years" around 1970, when a period of extreme cooling almost devastated his country's economy."
This was reported by NewsMax under the headline:
"Temperature Data Being Faked to Show Global Warming"
The source, of course, was Paul Homewood. Euan Mearns chimed in with:
"In this post I examine the records of eight climate stations on Iceland and find the following:
There is wholesale over writing and adjustment of raw temperature records, especially pre-1970 with an overwhelming tendency to cool the past that makes the present appear to be anomalously warm."
So what did I find? Nothing that could be considered 'systematically "adjusted"'. There were differences, mainly with a clear reason. I'll list the eight stations below the jump.
Clive's V1 is accessible here. V3 in CSV format is here (10Mb zipfile). I have put the extracts relevant to Iceland on a small zipfile here.
A point of comparison is with the IMO records here. These, however, are not entirely unadjusted. They can be useful for resolving differences.
- GRIMSEY,TEIGARHORN
These two of the eight are very simple. Exactly the same in V1 and V3. - STYKKISHOLMUR
This is a long record, 1620 months to 1990. Of these nine have been changed. Eight are sign changes, one is a minor change. In all cases, V3 and the IMO record agree. Clearly V3 corrects errors in the V1 draft. - REYKJAVIK
This is also a long record. There are a lot of apparent changes. However, the real situation is revealed by a table of their magnitudes, in the top row, vs number of occurrences in the bottom:
Size 0 0.1 >0.1 Number 864 211 3
Almost all changes (211) are by ±0.1. That is, a rounding difference, presumably from monthly averaging. - HOFN I HORNAFIRDI and AKUREYRI
Again, a fair number of rounding differences, but at most four larger (>0.1) month changes in a long record - KEFLAVIKURFLU...
This actually had quite a lot of small changes - 159 in a 461 month record. But I found what is happening by looking in ver 2, which records duplicates. These are different records for the same location, but may be different equipment or measuring points. The V3 version and V1 version are both recorded as duplicates in V2. In V3, they chose whichever seemed best. - VESTMANNAEYJAR
At this stage, I have run out of investigative zeal. Here's the frequency table
0 0.1 0.2 0.3 0.4 >0.4 1050 37 28 20 11 4
There are 100 discrepancies in 1150 months, small, but several bigger than rounding. No obvious reason.
It is clear that there is no "wholesale over writing and adjustment" in the GHCN V3 unadjusted file for Iceland.
Early note on April 2015 global
Most of the NCEP data for April global surface temperature is in. It was the coolest month so far in this warm year. As is common lately, it started cool, and only really warmed up to average (relative to current warmth). It was however, a bit warmer than last November, when GISS reported 0.63°C. March was &0.84°C. My guess for GISS in April is a bit over 0.7°C.
Subscribe to:
Posts (Atom)