"The GWPF paid Terence Mills, professor of applied statistics at Loughborough University, £3,000 to write the report."
Well, those are cogent criticisms. But time series analysis is a respectable enough endeavour, so I read the report to see if the GWPF got its money's worth.
The answer is no, or maybe from their poiunt of view, yes. There are about 33 quite well-written pages, borrowing I suspect from lecture notes (nothing wrong with that). But the forecasts themselves are worthless. Gavin Schmidt tweeted the following test of the HADCRUT forecasts, which were from 2014, after just one year:

The thin blue line is 2015 information added by Gavin, and as you see, they are already outside the confidence intervals.
Now it might be said that briefly going beyond the CI's is note a complete refutation, though it very likely won't be brief. The real reason that the forecasts are worthless is that in every case they simply foretell that the expectation for the future is no change at all. The forecast is just constant after a few months, based on a very recent weighted average of data points. It has to be - that is built into the models. In fact, the author actually says this:
"The central aim of this report is to emphasise that, while statistical forecasting appears highly applicable to climate data, the choice of which stochastic model to fit to an observed time series largely determines the properties of forecasts of future observations and of measures of the associated forecast uncertainty, particularly as the forecast horizon increases."
It would be good if he had emphasised that more; it's true (my bold). But in fact what is emphasised is the forecast. And in the press, the slow growth of the forecast. But, as said, that is built in. His models are simply incapable of forecasting change. I'll show why.
You can see from the time series plot of HADCRUT 4:
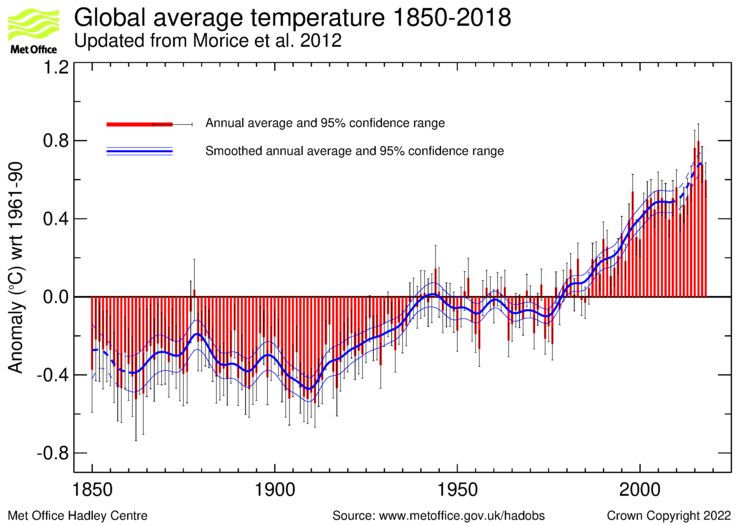
that especially over the last fifty years, forecasting on the basis of nothing changing would have been very unsuccessful. And there is no reason to expect it to be better in the future. So what gives?
Mills does two HADCRUT forecasts, as shown in Gavin's annotated plot above. One is a fit to the whole period, and one is a piecewise fit, in segments of which the last starts in 2002 (diagram below). For the whole period fit, he chooses a ARIMA(0,1,3) model:
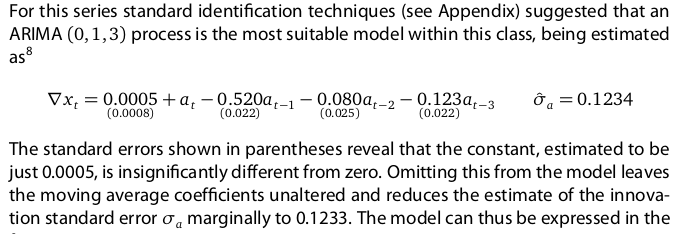
The a_t terms are iid random variables with the stated σ. I see no benefit in the extra complexity, but that is what he did. Now the key is the words "Omitting this from the model...". The constant term (drift) 0.0005 is the term that provides the trend. It is about 0.6 °C/Century - about what most people find for trend since 1850. And if you use just about any linear regression model, it will say that the trend is highly significant. I got a t-value, with AR(1), of 51. Yet he says it is not significant, and sets it to zero. And it is that setting to zero which guarantees a zero trend of the prediction. If he had left it at 0.6 °C/century, that would have been the predicted future trend. He could have set it to 1.2; that is at least as likely as zero.
So why does he get such little significance? The reason is that he differences, and then allows a different distribution (ARIMA) for the residuals. When summed again, the errors are now modelled as a random walk (with ARIMA modelled steps), as he explains. And it is far easier for a random walk to emulate a trend. His model has far less ability to discriminate trend value.
There are two things badly wrong with this:
- A random walk is unbounded, and is unphysical. Over time, temperatures can go anywhere, and will with eventual probability 1. They will surely go below 0K, and above 100°C. That hasn't happened. Now some may say, well, maybe the random walk is only for a finite period. But that is no use for prediction, if you don't know when the period is. And anyway it is highly implausible that the mechanism would suddenly change.
- A random walk is a solution to a stochastic de. The future is determined principally by the current state. But there is no reason to think that random fluctuations of weather would behave that way. A heat wave doesn't establish a new base point. We know that there is physics that brings it back to equilibrium (eg S-B).
Also bad is that he uses one model for the whole range to predict from 2014. But clearly things have changed since 1850. To remedy this, perhaps, Mills also offers a segmented model:
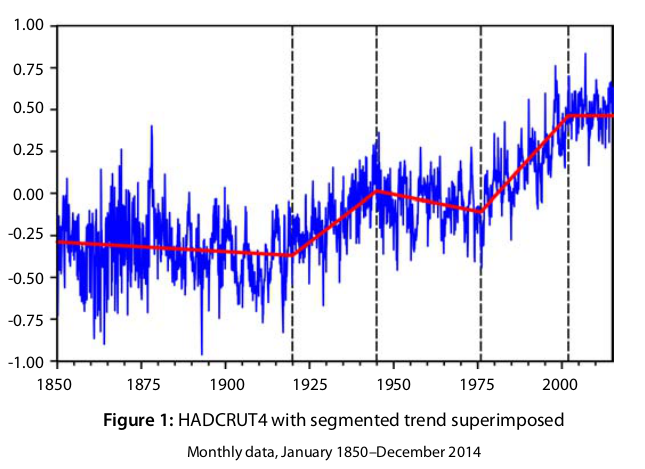
This time he uses an autoregressive model, AR(4), for the residuals of a linear regression. So at least the residuals aren't modelled as a random walk. He uses the final segment 2002-2014 for the prediction, and says of it that the slope is 0.8°C/Cen, and the t-value relative to 0 is 1.26. So again, on this basis he omits the trend term. This again has the effect of treating it as certainly zero. But because of the short interval, the trend was both positive (best estimate) and very uncertain. If he had made it the observed 0.8, this slope would have carried through to the prediction, and within the 95% limits it could have been as high as 2.0 &C/Century, which is about what GCMs predict for the next few decades.
Conclusion
I come back to what he said:"the choice of which stochastic model to fit to an observed time series largely determines the properties of forecasts"
It does. And the trendless future is entirely determined by his decision to take the observed positive slopes and replace them with zero, with no uncertainty. This arbitrary and baseless decision is the entire basis for his no-growth forecasts. The forecast method itself is primitive - it simply projects to the future from a weighted average of a few of the most recent data points.
Update. I've been able to emulate the HADCRUT forecast process. The ARIMA forecasts, with no "omissions", are virtually identical to what you would get by projecting a straight line OLS regression slope over the same interval, shifted to start from some smoothed value of the endpoint. The only "improvement" here is that that linear extrapolation has its slope set arbitrarily to zero. I hope to blog the calculations tomorrow.
TL;DR: "If we remove the past trend from our statistical analysis, the resulting forecast is flat!"
ReplyDeleteI see you are no longer making some of the accusations you levelled at Prof Mills at WUWT. However, you completely mistake the purpose of his work, which is to explore the statistical behaviour of the HADCRUT and RSS data over their respective histories (up to end 2014). There is no attempt at a physical model at all - any more than there is in oil blending equations that have been developed empirically - e.g. http://www.lube-media.com/documents/contribute/Lube-Tech093-ViscosityBlendingEquations.pdf - any more than your linear model is based on anything physical. Indeed, back projecting your linear model at 0.6 degrees per century implies temperatures below absolute zero less than 50,000 years ago.
ReplyDeleteUsing the gretl package, I find a linear model on the data from Woodfortrees for HADCRUT4
Model 10: OLS, using observations 1850:01-2014:12 (T = 1980)
Dependent variable: HADCRUT4
coefficient std. error t-ratio p-value
---------------------------------------------------------
const −0.507785 0.00891402 −56.96 0.0000 ***
time 0.000399381 7.79480e-06 51.24 0.0000 ***
Mean dependent var −0.112198 S.D. dependent var 0.302357
Sum squared resid 77.74100 S.E. of regression 0.198249
R-squared 0.570300 Adjusted R-squared 0.570083
F(1, 1978) 2625.213 P-value(F) 0.000000
Log-likelihood 395.5964 Akaike criterion −787.1928
Schwarz criterion −776.0111 Hannan-Quinn −783.0850
rho 0.753234 Durbin-Watson 0.493833
where the time increment is 1 per month - it suffers from serious levels of autocorrelation, as indicated by the DW value, and the standard error is over half the inter quartile range of the data. The high t values for the regression coefficients are thus spurious.
For comparison, the ARIMA(0,1,3) model produces
Model 7: ARIMA, using observations 1850:02-2014:12 (T = 1979)
Estimated using Kalman filter (exact ML)
Dependent variable: (1-L) HADCRUT4
Standard errors based on Hessian
coefficient std. error z p-value
------------------------------------------------------------
const 0.000534454 0.000783477 0.6822 0.4951
theta_1 −0.517842 0.0225032 −23.01 3.54e-117 ***
theta_2 −0.0802394 0.0259927 −3.087 0.0020 ***
theta_3 −0.119860 0.0232144 −5.163 2.43e-07 ***
Mean dependent var 0.000673 S.D. dependent var 0.139316
Mean of innovations 0.000238 S.D. of innovations 0.123333
Covariance matrix of regression coefficients:
const theta_1 theta_2 theta_3
6.13837e-07 5.53465e-08 2.39223e-08 1.18355e-07 const
5.06394e-04 -2.86922e-04 -1.95562e-05 theta_1
6.75618e-04 -2.29751e-04 theta_2
5.38909e-04 theta_3
Removing the constant produces
Model 12: ARIMA, using observations 1850:02-2014:12 (T = 1979)
Estimated using Kalman filter (exact ML)
Dependent variable: (1-L) HADCRUT4
Standard errors based on Hessian
coefficient std. error z p-value
---------------------------------------------------------
theta_1 −0.517562 0.0224786 −23.02 2.64e-117 ***
theta_2 −0.0800007 0.0258845 −3.091 0.0020 ***
theta_3 −0.119490 0.0231740 −5.156 2.52e-07 ***
Mean dependent var 0.000673 S.D. dependent var 0.139316
Mean of innovations 0.002123 S.D. of innovations 0.123348
These models both account better for the variations in the data, and are thus superior descriptors of it. The exclusion of the constant trend term is not to be decried - it is an act of parsimony which has no substantive effect on the model error. Many climate models omit variables that have a clear physical basis for influencing climate because there are difficulties in calibrating them with the rest of the model framework: they add nothing to the framework.
"I see you are no longer making some of the accusations you levelled at Prof Mills at WUWT."
DeleteWUWT comments are here and here. Basically the same. It's true that I haven't pursued here the issue of joint distribution of the parameters. I think it is still valid, but the joint variation isn't the cause of failed significance. On examination, it is the random walk modelling.
"However, you completely mistake the purpose of his work, which is to explore the statistical behaviour of the HADCRUT and RSS data over their respective histories (up to end 2014). "
The title of the paper is:
"STATISTICAL FORECASTING
How fast will future warming be?"
It's all about forecasting. The sub-heading in the Express said
"GROUNDBREAKING new research suggests the global average temperature will remain relatively unchanged by the end of the century."
The Mail:
"Is the world really warming up? Planet may be no hotter at the end of the century than it is now, claims controversial report"
Murdoch takes a different line, but again long term forecasting:
"British winters will be slightly warmer but there will be no change in summer,"
GWPF, which commissioned it, uses the title as headline, with the sub-head:
"A new paper published today by the Global Warming Policy Foundation explains how statistical forecasting methods can provide an important contrast to climate model-based predictions of future global warming."
It's all about forecasting there.
"it is an act of parsimony which has no substantive effect on the model error."
But it has a very substantive effect on the trend of future forecasts - in fact guarantees zero. If you resisted that parsimony, in the segmented case, you would have a 0.8°C trend. If you replaced it with 1.6°C, equally valid, you would have very close to the near future IPCC trend. And of course you would soon break through his ridiculous CIs.
"any more than your linear model is based on anything physical"
I don't advocate a linear model. I said that the trend of 0.6°C/Cen is not a good basis for prediction. The temperature is obviously not behaving linearly. I do think that a reasonable model is some secular variation with ARIMA noise, though even then the noise is obviously part cyclic. I've dealt with some of that here.
I provided the correlation matrix for you to comment on, which you omitted to do.
DeleteHow newspapers report is not relevant to the content of the paper: there is plenty of misreporting (usually alarmist, not supported by the research) encouraged by the need for clickbait.
The forecasts are not single valued: they are for a range with a 95% confidence limit. That data with high levels of autocorrelation shows some successive points that lie outside the band should not be a surprise. This is easy to see by re-estimating the model for 1850 to end 1996, and noting that the subsequent El Niño produces several out of band data points, before the subsequent data continue to lie very comfortably inside the bands - see graph below
http://a.disquscdn.com/uploads/mediaembed/images/3286/3886/original.jpg
But what is then very bad is that, by omitting the constant, he treats it as zero
vs I don't advocate a linear model OK, which is it? Very bad not to have a linear model, or not recommended?
I estimated the model as ARIMA (0,1,4), finding that theta_4 was significant at the 10% level, while the constant was again not statistically significant. On your version of things, you would retain the constant in preference to theta_4. Prof Mills ditched both, as would I. And it is far easier for a random walk to emulate a trend. That's the point. It's a better description of the data.
Link to chart if HTML allowed
Delete"I provided the correlation matrix for you to comment on, which you omitted to do."
DeleteI'm not sure what comment you expect. I queried where the sd came from on which he dismissed the trend. It looks like it's just the sqrt of the diagonal value of covariance. Is that OK? In practice, it may not make much difference, but it's not exactly textbook.
"How newspapers report is not relevant to the content of the paper"
They'll report what GWPF is telling them. But you can't evade responsibility for the title of the paper.
"The forecasts are not single valued: they are for a range with a 95% confidence limit."
Yes. And your linked plot shows that that is a wide range. A rise of 1°C since 1996 would be within the bounds. So the forecast is, probably continuing about 1996 values, but could be anything. Not so informative.
"Very bad not to have a linear model, or not recommended?"
Not an option. He has a linear model for forecast. He just set the trend to zero.
"On your version of things, you would retain the constant in preference to theta_4. Prof Mills ditched both, as would I."
Ditching theta_4 has virtually no effect on the forecast. The trend does. It's what most people would like to know.
My basic objection is this. He creates a model in which the trend, or drift, is very uncertain. Then he says, OK, it could be zero, let's say zero. But then he omits - so it is zero with certainty. The large uncertainty that allowed him to ditch is then excluded from the CI calculations. That's just wrong.
The CI's are already so wide that long term forecasts are fuzzy. If you add the uncertainty of the trend, it's worse again.
Here is my version of your post-1996 plot. I'm writing a post based on my emulations, using the R arima package.
Similar considerations apply to the model broken into sub periods. Of course, most of the sub periods have significant trend constants - only the most recent period shows no trend. Similar conclusions can be reached by examining an 8 year centred moving average.
ReplyDeleteThe forecast periods presented are modest - only out to 2020, and presume no change in behaviour. However, the segmented analysis demonstrates that there are turning points. The fact that we already have a couple of subsequent data points that lie outside the band within which 95% of observations are expected to lie is not a useful criticism of the forecast - at least until there is a much more data in that region. After all, the linear model has rather more points outside its confidence bands already.
"The forecast periods presented are modest - only out to 2020"
DeleteAs a short term forecast, as Gavin noted, it failed within the year.
"However, the segmented analysis demonstrates that there are turning points."
The forecasts make no provision for change of trend. They allow no trend.
The Schmidt critique is simply inane...as in "doesn't even understand the model enough to critique it" levels of inane. In fact, if you extend it to Feb. we're already back inside the confidence band. Which is about what you'd expect for 13 months of obs. on a 95% confidence interval where there's also an El Nino effect that's likely to produce outliers just as it did in 1997.
DeleteLaugh all you want at Mills' model though. Because it's really the statisticians who are presently laughing at those of you who reside in the climate community & resist the use of well established mainstream forecasting methods for your fields.
"In fact, if you extend it to Feb. we're already back inside the confidence band. "
DeleteWhere on Earth do you get that from? HADCRUT results for February won't be out for weeks. When they do emerge, they will show a huge increase on January.
IID,
DeleteApologies, I see that you meant including January, which was indeed lower. But Februaru will be back well outside the band.
Your january shows a huge increase on december but the HADCRUT shows january was down from december.
DeleteI have to express a certain grudging admiration for IDAU's willingness to vigorously defend a "forecast" that is simply laughable.
ReplyDeleteThe forecast is no more laughable than many (most?) that have emerged from the climate science community, and the contortions to deny the consequences of basic statistical procedure are a wonder to behold.
DeleteThis statistical forecast has confidence limits (when starting in 1996 like you do) bigger than the range for hadcrut4 from the beginning of the series in 1850.
DeleteA wonder to behold indeed. High quality forecasting.
A wonder indeed
DeleteMuch narrower spread there.
DeleteMust be bad of course. Widen them like Mills. The true mark of a good forecast.
Somewhat that does add up. Fore some.
IDAU,
Delete"A wonder indeed"
OK, here is the same plot with the ARIMA(0,1,3) forecast superimposed. That is with Mills' CI's, which are actually far too narrow, and do not take account of trend uncertainty. The spread is far greater than the CMIP forecasts, and actually embraces the RCP2.5 and maybe 4.5 results.
The more I research these climate indices, the more
ReplyDeleteI think they can be analyzed as deterministic rather
than statistical time-series. That would be a great
advance for no other reason than to get the
uncertainty monkey off our backs.
I have been harping on the QBO index, which is a
highly deterministic signal, in spite of what
has been written on it.
http://imageshack.com/a/img922/2119/ea0m2v.gif
Lindzen must have studied the QBO for 50 years
without finding the synchronous forcing mechanism
that drives it. The fact that he spent that
long should afford us to spend a few months talking
it up and getting others excited about it. And that
should promote interest in ENSO which I am also
convinced has a similar synchronous forcing
mechanism, albeit obscured by other noisy factors.
If that happens, these forecasts will become much
more solid, and everything will add up .. much
to the chagrin of those that don't want it to add up.
Incredible forecast. It looks like an extrapolation from a flat trend... This is nonsense. Here is a forecast from Met Office : http://www.metoffice.gov.uk/news/releases/archive/2016/decadal-forecast.
ReplyDeleteThe Met Office forecast is for moving annual average temperature anomalies, not monthly temperature anomalies. The Met Office longer range forecasting (seasonal and longer) does not have a good track record - so much so, they withdrew from publicising their forecasts for several years.
DeleteSo far the Met Office is more on track than Mills' forecast.
DeleteBut of course. That forecast does not have the true mark of a good forecast: HUGE CI. Mills' must therefore be better.
Smith et al is there for all to read. It is among the first attempts to do decadal forecasting, and any fair evaluation of it, as a very early attempt at what was thought to be impossible, is that it was a pretty good forecast.
DeleteOur system predicts that internal variability will partially offset the anthropogenic global warming signal for the next few years. However, climate will continue to warm, with at least half of the years after 2009 predicted to exceed the warmest year currently on record.
It "paused". Of 2010, 2011, 2012, 2013, and 2014, two were warmest years. Followed by 2015.
Indeed Met Office is forecasting annual means but the likelly range for 2016-2020 shows 0.7-1.2 above 20th century. So the lower range, 0.7, could never contain monthly anomalies as low as the ones showed in Nick's article, I think.
DeleteAs for one year forecasts, Met Office always falls in the estimated range.
Funny, considering that Mills anticipated all of the foregoing frivolous charges on his model.
Delete"The models considered in the report also have the ability to be updated as new
observations become available. At the time of writing, the HADCRUT4 observations
for the first four months of 2015 were 0.690, 0.660, 0.680 and 0.655. Forecasts from the
ARIMA (0, 1, 3) model made at April 2015 are now 0.642 for May, 0.635 for June and
0.633 thereafter, up from the forecast of 0.582 made at December 2014. This uplift is
a consequence of the forecasts for the first four months of 2015, these being 0.588,
0.593, 0.582 and 0.582, underestimating the actual outturns, although the latter are
well inside the calculated forecast intervals."
Yeah! That's the ticket!
Delete(1) Omit trend
(2) Use astronomically huge CI intervals on trendless prediction
(3) Update forecast with built in actual future temperature values
(4) Forecast becomes never ending hindcast with astronomically huge CI intervals
One would normally call that a nocast, you heard it here 1st, uncast.
Yeah! That's the ticket!
In other news, the RSS TMT "gold standard" has been "altered" (in Lamar Smith lingoland).
Reason for the change? Monetary inflation as uncast/nocast from econometrics.
But here's the very best of all nocasts/uncasts, Deniersvilly will use whichever temperature time series index shows the lowest XX (or even X) year trend.
Monkers will now use UAH v6.0b666, UAH tries to match the "unaltered" "gold standard" but RSS has "altered" the "gold standard" without government supervision even.
UAH is guilty as charged of "fixing" the "gold standard" and I think we now need a Congressional hearing and legislation to "fix" the temperature time series indices to their "fixed" "gold standard" or some such.
ehak:
ReplyDeleteIsn't it a little early to tell? The MO forecast was only published on 1 February, and benefits from using a further year of data, and in any case is only projecting moving annual average temperature anomalies.
No. Sure is better than Mills. Unless you use wide CI as a quality criterion of course.
DeleteYou value being wrong with more certainty highly.
DeleteArguing the fine points of statistics with someone whose personal Bayesian prior is independent of whether energy is conserved is unlikely to be fruitful.
ReplyDeleteMills wouldn't put money on his forecast. Would you? http://julesandjames.blogspot.com/2016/02/no-terence-mills-does-not-believe-his.html
ReplyDeleteSo that's how we settle scientific disagreement these days, eh? By betting on them and declaring "victory" when the proposed bet is dismissed as a childish antic?
DeleteWould you take the bet? You would be foolish to bet on Mills.
DeleteWagering on your science or promising prize money to refute it is a tell-tale sign of a crackpot.
ReplyDeleteOne should occasionally revisit John Carlos Baez's Crackpot Index,
especially if you are going to crow about some achievement:
http://math.ucr.edu/home/baez/crackpot.html
I think James Annan made a mistake in offering a bet.
On the other hand, he's makin' bank: http://julesandjames.blogspot.com/2016/01/happy-10987654321.html
DeleteNot exactly. His highest profile bet to date was on the losing side. http://wattsupwiththat.com/2012/01/13/the-standstill-not-the-increase-is-now-this-warm-periods-defining-characteristic/
DeleteThe £100 bet is of higher profile than the $10,000 one? Or the £666 one? If so, does that somehow mean he's not makin' bank?
Delete